This week I’ve been challenged to think about ‘future trends’ by eminent futurologist Bryan Alexander. In advance of our live chat about this, I’ve brought forward a post about risks I foresee from generative AI to some of the knowledge economies that matter to higher ed. This is the third in my ‘risks’ mini-series: the others looked at risks to students and to the work of teaching.
If you’re new here, you’ll have worked out that I’m not a big, season-ticket fan of the generative AI circus. I try to base my concerns on really existing harms, and on the history of ‘Artificial Intelligence’ as a project in and out of education. But with Bryan’s encouragement, I’ve taken a bold peek into the 'future’. This post is one of my more imperfect and will definitely need some running repairs, but I wanted to get it online before my chat with Bryan. I know the collective intelligence of Bryan and his audience will challenge me to improve it.
Not those risks…
Compared with the risks of ‘generative AI’ that I’ve banged on about before – really existing harms to workers and creative artists, writers and students, teachers and researchers, and to everyone who is a data subject under surveillance – the risks to ‘knowledge’ seem a bit obscure. Speculative, even. The last thing I want is to take attention from those real harms with some fantasy about human knowledge or human ‘thought’ being overthrown by machines. Versions of this fantasy are tediously familiar by now. It’s almost a religious dogma in Silicon Valley, linking accelerationists and doomers: the accelerationists rushing with open arms towards the mind-machine rapture, while the doomers see nothing but killer robots on the same horizon. (Killer robots have already arrived for some people, of course, but the white men who pass as philosophers in Silicon Valley are not their targets at the present time).
Readers, as you know by now, I’ll take some convincing that a ‘general artificial intelligence’ is around the next corner, or that any computational system has agency of its own. I’m here for the risks and harms to actual people, especially from the hugely unequal agency that people have in relation to technical systems. But I think questions about knowledge – that is, about practices of knowledge production and economies of exchange – are deeply connected with the more direct harms that I’ve written about. And universities, with their special values and practices and resources of knowledge, have a unique responsibility here. How universities value teachers and their know-how, for example, impacts on how digital platforms are allowed to restructure the work of teaching. How synthetic media are integrated into learning and assessment is shaped by values universities have around students and their learning. And the space of public discourse looks very different depending on how universities define and defend their role there.
Universities also provide research and innovation to the tech sector, despite the brain drain that has dramatically reduced capacity for building alternative ecologies:
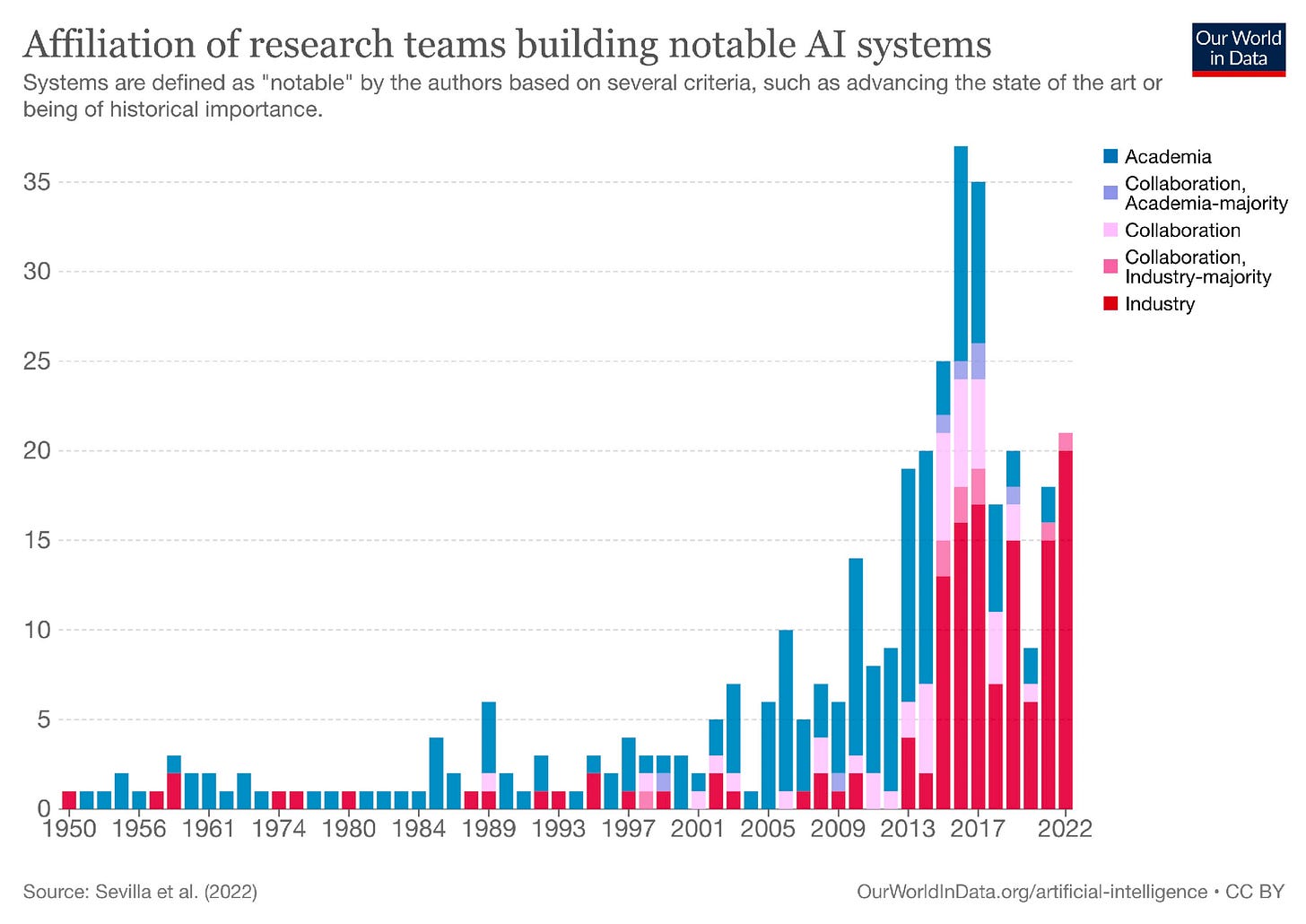
A trajectory that coincides closely with the influx of venture capital to the generative AI sector:
Still, universities are among the very few places outside of big tech where there is the expertise to make policies, provide governance, and explain the ethical issues involved in generative AI. And yes, shape knowledge economies. It matters how universities respond. And we don’t have to believe in the AI singularity or think generative text is rewiring our brains to worry about what is happening to the very real academic economies of teaching, research and public communication.
The last knowledge revolution
Fans like to compare generative AI to the print revolution (if they manage to avoid more impressive comparisons, like the discovery of fire). But there is a far more recent paradigm shift that we are still living through: the rise of the internet, and its capacity for fast, cheap, frictionless distribution of content. In some ways synthetic media can be seen as intensifying internet effects. In other ways they counter and might even reverse some of the effects we have got used to. But I think this is the right context to think about the impacts of synthetic media.
It allows us to use some of the same insights and theories we have developed for understanding networked information, seeing its effects as economic and socio-cultural as well as technical; seeing them as extending far beyond the immediate user experience; seeing them as systemic, though sometimes unpredictable and chaotic (I think ‘emergent’ is the preferred term).
The changes in knowledge practice have been profound, but more gradual than the champions of the new have predicted. Of course, education has had its own dot com bubbles, its snake oil sellers, its digital diploma mills. The death of mainstream higher education has been foretold often, and gleefully. Remember when the assassin was interactive television? YouTube.edu and iTunesU? Self-organised learning environments? MOOCs? But the mainstream sector lives on, it just does things differently.
It’s for other posts to explore what it means for individuals to read, write and research differently. Here I’m thinking about the regimes and infrastructures that shape practice and how they have changed.
I know I bought into the dream of a connected world in which everyone would have access to the kind of knowledge and knowledge-building that goes on in (our best version of) the university. Networked, connected and open learning were HE’s contribution to the dream. Then, as social media came to dominate, and ‘online’ also meant cyberbullying, extremism, scams and porn, compulsive clicking and shortened attention spans, universities developed resources to support students with all of that. They also developed safe, relatively closed online spaces to teach in, and ways of facilitating them (let’s call this response 2.0). It allowed universities to rise to the challenge of the global pandemic.
Then, as those systems and spaces were consolidated into platforms, universities had to respond to the impact of data everywhere, of hugely powerful corporations managing the services that they depend on (like publishing, and search, as well as teaching), and surveillance as a core business model. We are now well into response 3.0.
That universities have adapted should make us confident that they will adapt to synthetic media models as well. But adaptation is never without some cost to the organism. As a reliance on digital content has grown, universities have had to invest more and more to deal with unwanted effects, whether that means new ways of supporting staff and students, investment in digital platforms and infrastructure, IT support, security and privacy services, or subscriptions on increasingly poor terms.
While teaching and research continue at a greater scale and volume than ever before, scale and volume present their own problems, to which data-based platforms and algorithmic processes are always on hand to provide a solution. More investment in algorithmic efficiencies means paying fewer staff to work harder, to teach more students, or to produce more research papers and patents. In the face of competition from tech-based businesses – in online teaching and accreditation, for example, or research and innovation services, or (especially) in public communication of ideas - universities have opted to become more rather than less like those tech-based businesses In response 3.0 mode, I’m not sure universities have been so good at protecting students from platform logics, or the general ‘enshittification’ of the internet. Universities still have agency and expertise, for example in relation to specialist platforms and services, but increasingly less collective will against the efficiencies of outsourcing and the concentrated power of commercial platforms.
Continuities and disruptions
As the synthetic media circus arrives in this landscape of ongoing adaptation, what discontinuities can we expect, and what chaotic and novel effects? Here are some of my thoughts. You will almost certainly have your own.
Despite platform dominance, the internet itself has no central ownership or control. Large media models are owned and controlled by large tech businesses: the only ones with the human and computational resources to build them. A transformer model is not a public commons with open standards and shared protocols but a closed, proprietary data structure with its own secret system of weights and parameters and data sources.
The internet is distributed. Internet-compatible content and apps can be run on almost any hardware. Synthetic media models are located in custom server farms. They can be run, at the present time, only on one kind of GPU (chips made by Nvidia). The links between Nvidia, Microsoft and OpenAI mean that at the present time what is called ‘generative AI’ is pretty much a born-monopoly.
Before platformisation there was a vast flowering of experimentation in internet content, services and interfaces. Also in business models (many failed, of course). With large language models, the platform came first. Experimentation is taking place around the existing models, but very much in start-up mode. Everyone is waiting for the big buy-out. Few are looking to build credible, long-term alternatives (except for a few public projects at the European level, that I mentioned in my last post). And they all need server space on those same Nvdia GPUs (did I mention that they also have their own, unique, development environment, CUDA?)
Media for online distribution can be produced in a wide variety of ways. Born digital or digitised, coded, remixed and linked, produced solo or in collaboration, created with a blend of digital and analogue tools. Synthetic models produce media one way only: by inference from a data model. User input is currently limited to the prompt window, though we can expect that to be replaced with even more frictionless interfaces in time.
The internet supports a ‘long tail’ user profile so that rare, minority, outlying and countercultural content can flourish with small audiences - though platformisation has certainly pushed this into marginal spaces. Transformer models define content in terms of norms; they are oriented to the content that meets the majority of user needs.
Internet knowledge is infinitely extensible, just like the network itself. Add another server, web site, app or service, and shared protocols mean your content can be found. Content on the internet is biased by production resources but it is still possible, with political will and resources, for minority language and cultural resources to be produced and have an impact. Models are integrative. New content must be processed and rendered statistically, in relation to existing content, before it can be used. Synthetic media are locked into the past, the unequal history of content production, and the historic biases of that data.
Search is certainly secretive, and also biased. However, search results point at sources of information and content that really exist in the network. Users can choose where to look. Inference produces results that may not exist in the original training data, may not be referenced, or may point to non-existent or spurious sources. Online information may not be ‘true’, but it is ‘real’, can be found, and its authority looked into.
Obviously this is a selection. I’ve leaned into the continuities to counter the narrative of a sudden ‘rupture’: the world transformed. But it does feel to me that large media models, now they are being integrated into the online information system, are likely to amplify some of its more harmful effects. Particularly the concentration of market power and data in big platforms, owned and controlled by a very few, very rich people, who stay that way only if they can persuade the rest of us that something called ‘generative AI’ has actual value. To the concentration of computational capacity and data we we can now add the capture of content as a third mode of big tech empowerment. How might this play out in the knowledge economies that universities and their members have come to depend on?
Capturing content
Early in the generative AI hype and response cycle, Naomi Klein described it as:
the wealthiest companies in history (Microsoft, Apple, Google, Meta, Amazon …) unilaterally seizing the sum total of human knowledge that exists in digital, scrapable form and walling it off inside proprietary products
As I prepared for my chat with Bryan, it seemed that some people at the Senate Judiciary Hearings on AI were having issues too. We’ll not mention that ‘regulators abroad’ (the EU) and very many think tanks and academics (like Naomi Klein) and indeed unionised workers have answered this question, but latecomers are always welcome to the party.
On the internet, content is proverbially king. With large media models, that content is sucked into privately owned servers and data stacks as ‘training’. This puts the big tech firms into direct contention with existing legal frameworks around copyright (and in this case we do need to look back to the print revolution to appreciate how well established they are, and how critical to the economy of text production). Big tech makes no secret of the fact that they want to bring this structure down. Fed up with the costs and uncertainties associated with fighting separate copyright cases (that they may well lose), they have decided to go for the whole legal edifice. As the LA Times reports today, they are going straight to governments to plead their case:
“Because copyright today covers virtually every sort of human expression — including blogposts, photographs, forum posts, scraps of software code, and government documents — it would be impossible to train today’s leading AI models without using copyrighted materials,” OpenAI argued in its submission to the House of Lords
LA Times 12/01/2024
Of course I hope for good conclusions from the White House and from the copyright lawsuits being brought by the representatives of artists and writers. But the Goliath is vast, the law moves slowly compared with technology in the middle of a venture capital boom, and big tech has now signalled its intent to bypass the law in its direct appeal to governments. In the UK, unfortunately, I have no confidence that our government will stand up to the allure of becoming an ‘AI global leader’; our nation’s really existing cultural resources and creative sector has little chance against this chimera.
The future of your and my access to online content now depends on the stories big tech tells to governments, and partly on the deals they do with big content providers. We don’t yet know exactly what these deals might look like, but we do know that the future of knowledge production depends on it. In academic publishing, Clarivate—which owns Web of Science and ProQuest—has announced a partnership with AI21 Labs. Elsevier is pioneering an AI interface on its trusted content. Trusted, that is, thanks to peer review by unpaid academics. Springer has Dimensions AI. These in-house developments are small in scope so far, which is one reason to think that partnerships with big tech might be unavoidable, just for the functionality.
The generative models need content, but thanks to the massive popularity of the models as natural language interfaces, they can pressure content providers to come to a deal. Who wins here will depend on who has the best story about value, power, and futures. And I’m afraid that individual producers will lose. Journalists, film- and music-makers, writers and academics with new work to promote will be competing for audiences with endless remakes of their own (and everyone else’s) Greatest Hits. It also seems fairly certain that readers will lose, especially readers who are used to relatively low-cost access to trusted content.
As I was preparing this post, Jeff Pooley published a brilliant article about the impact of generative AI on publishing that covers everything I wanted to say about the industry, and more. So I’m mainly going to share a couple of his takes, and recommend you read the original. Pooley takes off from the NY Times lawsuit against OpenAI that I trailed back in August. Like me, he is cynical about the agenda behind this. Rather than slaying the generative AI dragon in order to defend the rights of journalists and creatives, ‘big news’ might just be positioning itself for more favourable terms from ‘big tech’. Like academic publishers, the NYT itself reports rather coyly that news publishers are ‘in talks’ with the model providers about exactly how their businesses can partner to capture the market in stuff that people need to know.
Pooley:
Thus the two main sources of trustworthy knowledge, science and journalism, are poised to extract protection money—to otherwise exploit their vast pools of vetted text as “training data.” But there’s a key difference between the news and science: Journalists’ salaries, and the cost of reporting, are covered by the companies. Not so for scholarly publishing: Academics, of course, write and review for free.
Indeed we do, or rather, universities foot the bill, just as they fork out for the books, e-books and e-journals they’ve paid their employees to produce, and again for the right to licence that work for open access. The business model for news is notoriously fragile, while the business model for academic publishing seems in robust health, thanks to the structure of academic reward. Universities gave up their capacity to do anything about this when they sold off their publishing presses and in-house journals, and of course they now depend on a datafied publishing industry for all the metrics that govern academic careers.
Pooley notes that both big tech and big publishers:
extract data from behavior to feed predictive models that, in turn, get refined and sold to customers. In one case it’s Facebook posts and in the other abstracts and citations, but either way the point is to mint money from the by-products of (consumer or scholarly) behavior.
So this vision of the future has academic publishers - already major extractors of value from the university sector, already sources of data surveillance and inequity among universities and their members - getting into bed with even more predatory players.
Crap-ifying search
Generative AI inserts itself into every one of the trajectories I identified with the development of online platforms. In particular, as we’ve just seen, it provides a natural language interface on search - of closed as well as open data systems. In fact there is growing evidence that this is the main way it is being used. Search engines already incorporate language model interfaces - Bing Chat into MS Edge, Bard into Google services, though its Search Generative Experience seems to be where Google is most invested. And there are plenty of stories about how major content sites are watching their traffic drop off a cliff as Google search results morph into text summaries, including ‘snippets’ that mean users don’t have to click through to the source.
A team of Stanford University scientist recently evaluated four search engines powered by generative AI — Bing Chat, NeevaAI, perplexity.ai and YouChat — and found that only about half of the sentences produced in response to a query could be fully supported by factual citations.
We believe that these results are concerningly low for systems that may serve as a primary tool for information-seeking users,” the researchers concluded, “especially given their facade of trustworthiness.”
Search-engine results at least offer the option to click through to sources and citations. But increasingly, language models are being used as stand-alone sources of information. And below the surface of whatever interface users choose, content on the web is increasingly likely to have been written by bots anyway, or written by a person under such time pressure that text generation is the only way to keep their job. Thus generative AI is sucking on its own exhaust, as people paid to write summaries for training the next generation of models are using autogeneration to write them.
Auto-generate makes it almost cost-free to flood the public sphere with junk, propaganda and disinformation. Two classic reads on this topic are James Vincent’s AI is killing the old web the new web is struggling to be born, and Ted Goia’s Thirty signs you are living in an information crap-pocalypse.
This is how the Information Age ends, and it’s happening right now. In the last 12 months, the garbage inflows into our culture have increased exponentially … The result is a crisis of trust unlike anything seen before in modern history.
Ted Goia, June 2023.
Goia has fully inhabited the trajectory from nirvana to hellhole, and he continues to faithfully record the descent.
In hell’s latest offering. versions of ChatGPT that create fraudulent and phishing texts have tens of thousands of subscribers, while public figures spouting hate speech and non-consensual porn cann be generated with a couple of swipes by underage tiktoc users. Put the firehose of content together with the fact that users find it almost impossible to detect when text (or images, or moving images) are AI generated, and you have the perfect storm. All this in the year that almost half the world’s population will be voting for new governments (Bangladesh is one of the first). Enjoy your time on Reface, guys.
None of these new technical capabilities would matter as much if they were not being dumped into an online environment already tuned for disinformation and distrust. The purpose behind most online content, pre-generative text, was not to communicate ideas but to drive users’ attention, their behaviour and beliefs, to hook them and rook them, and meanwhile to scrape and sell their data. It’s the same dark money ideologues and pornographers who are now invested in generative AI video, just as it is advertising money that is behind so much generated text online. But advertising and influencer memes were already dominating the algorithms and the bandwidth. What has happened is that content production, a narrow point in the firehose, has been blown wide open.
The tech industry has always been reluctant to invest in content moderation and validation, but the generative boom makes this problem fundamentally intractable. Model training data is (for most models) a matter of huge secrecy, and most of the corpora ingested are themselves unregulated. Even if this were not the case, no reliable record exists of the tens of thousands of interventions made by data workers, or by the increasingly automated processes of refinement and reversioning. So the ‘content’ of foundation models is not something that can be examined, let alone regulated.
‘Check with other sources’
Of course we encourage students to look away from the shitshow that is online content and take their reading material from authoritative sources. But there is no firewall between the open internet of content and the sources students use for academic work. Many are not even aware when they are accessing paid-for library services via Google Scholar, for example. And many are now looking for academic content via ChatGPT style interfaces, especially those promising ‘detection free’ outputs. That students should do this is not incidental but essential to the generative AI business model.
The big tech companies have already managed to concentrate, accumulate, and leverage global data. Also computing power, and certain kinds of computational expertise. What they hope to leverage through the building of foundation models is the world of content, that is valued cultural knowledge. The knowledge held by universities and cultural institutions and academic publishers were vital sources for the foundational models, but now they are competitors and enemies. Students are by far the biggest users of this knowledge resource, and the habits they acquire as students continue with them through their (typically) knowledge-focused and high earning careers. So it is essential for the business model that students, in particular, stop looking for their content in these traditional places, and turn to the foundation models instead.
We have spent twenty years developing ways of helping students to navigate online information, and ten years dealing with the effects of social media on how students read and produce text. In many respects, academic practice has not fully negotiated these challenges. We are only starting to grasp what is involved in helping students navigate their relationship with generative text. As educators, I know we have amazing strategies. Working with students, we will find more. But as a sector, universities don’t seem to have grasped that we are facing a technology hype cycle that is targeting students in order to turn them away from using the knowledge and the knowledgable strategies we offer them.
According to an extensive research project by the LSE, there is already a crisis of trust in the written word, leading readers to experience: ‘confusion, cynicism, fragmentation, irresponsibility and apathy’. Now the plausibility of text and image generation threatens to further undermine our remedies against distrust, our measures for determining what knowledge counts as authoritative, and our advice to students to ‘read carefully’. Guidance from UNESCO, the UK Government, every university, and even OpenAI itself, is for students to ‘check with other sources of information’ before relying on synthetic text. But as synthetic text become the interface of choice for search, and as the results of search become more and more likely to be synthetic, it becomes difficult to see how this is going to work. Or at least, how students are supposed to operationalise this advice using their own tools and resources. What I think students may be hearing, from all the contradictory advice they are getting, is ‘keep trying until you get something that sounds right’.
Way back in February, as its first public outing, Bard gave a wrong answer to a question about a telescope, and shares plummeted. Back then, someone in the room checked the answer with Google. What happens when Google and Bard are one, or when the internet and large language models of it become indistinguishable to most users? When the models are checking their own homework? Perhaps we should to go back to an idea of learning that is filling students’ minds with the information that (we think) they will need. Then there might still be someone in the room who has memorised the relevant fact, and who is willing to stand up and say ‘No, it was the VLT/NACO telescope that took the first picture of a planet outside our solar system, and not the James Webb!’. There might be a filmic moment here, like the end of Farenheit 451 when we learn that all the drifters have memorised one book for posterity. But as a future for university learning, it feels as though quite a lot would have been lost.
On writing assignments and grading
Our hope is always that students will become writers, not necessarily to become great writers, or even to write as (part of) making a living, but to express their own meanings, in words that have been ordered and considered, as well as in unique images and voice recordings and created things. Writing and reading allow us to enter other people’s minds and opinions and worlds, sometimes at a great cultural distance to our own, expanding our ability to embrace difference and empathise with others. Writing creates a shareable reality, which is an important step towards a shared one.
But writing is hard work, and - except for a few ‘star’ turns - badly rewarded. Who is going to do the work of new writing if the rewards shrink still further, to match the costs of autogeneration? This is not a moral doctrine for writers to refuse auto-generative help (though some, like me, will continue to do this). It’s a worry about what I’m going to read in the future (and my students, too). It’s an economic reality that writing takes time, failures are inevitable and painful, and without some monetary reward, only the independently wealthy and the pathologically self-confident will ever embark on it.
Thousands of authors have signed an open letter condemning the negative impact of generative AI on publishing. These writers have already made their reputations and will, presumably, carry on writing, for a while at least, regardless of the changed conditions. Habits die hard. What incentive will there be for aspiring writers without a big name or established voice to acquire the habit? There are reports of established authors being ‘recommended to read’ AI rip-offs of their own books, and of best-seller lists containing over 80% bookspam. Amazon can’t or won' refused to label AI content on its publishing platform and has recently ‘dealt with’ the problem of bookspamming by limiting uploads to three books a day. I don’t know what kind of nootropics are available on Amazon, but if three books a day is normal for other writers, I’d like to order some.
One of the challenges of the internet for educators was that students had access to academic information independently of their course or their teachers. This led to much hand-wringing, but in the end a valuable reframing of the higher education offer. Away from universities being (only) a source of knowledge, and towards providing a space to develop conceptual frameworks and knowledgeable practices, for a lifetime of living and working in a connected world. I think it also led to a new appreciation of the value of authoritative knowledge, and of the expertise as well as the resources to be found in university libraries.
Now auto-writing is being promoted to students as a way of gaining independence from their teachers and their courses of study. But the sell is a different one. Students are not encouraged to learn differently but to see assignments as unreasonable demands on their time. Not to engage differently with the knowledge of their discipline, but to recycle a stale version of it until they sound credible. A thousand pop-up ads for ‘detection proof AI’ present the task of writing as ‘passing’ some kind of academic turing test. The sole function of teachers is to apply these tests. Teachers and their ‘AI detection powers’ are what stand in the way of the pass students deserve, enemies that can (luckily) be defeated with the ‘smart’ AI services that are just a click away.
These ads are not completely wrong. They are just ratcheting the economy of grades and grading a bit further along its present axis. Standardised questions can now be designed from AI-generated curriculum plans, with AI-generated marking rubrics, for AI systems to grade, while AI tutors offer to help students to perform as close to the AI-determined standard as humanly possible. Performance is everything. Where is the learner in this system? Her role is to be assigned a value – a grade – she can use this to pass into another arena of text production – from undergraduate to grad school, for example, or to writing 250 puff pieces a week. This mirrors other read-write loops online, where human beings are no longer required to engage in any meaningful way, but only to help push data and value around the system.
In this grading economy, if student assignments (however we define them) start to look more similar, what differences will matter? Should we focus our feedback and our grading on these differences, even if they come down to students’ different purchasing power in the marketplace of generative tools? Or should we be valuing them as differences – valuing what makes students unique, even if being unique means departing from the ‘norm’ that is referenced in the assessment rubric?
In the best version of this future, students might decide they have had enough of grades. Teachers might jump at the chance to stop grading and negotiate more meaningful relationships with students around their interests and the kind of knowledge projects they want to invest in. Instead of a normative assessment rubric, an eclectic range of examples. Instead of a test, tasks that students can choose or devise to showcase their abilities. Employers and professional bodies would find these portfolios of achievements far more informative than simple grades, could they detach themselves from the metrics of achievement long enough to appreciate them. But there is a big question mark over the business model of higher education if credentialising comes under threat. Ungrading is a wonderful pedagogical project, but universities still need to be there, holding open the spaces for teaching and learning in the fullest sense.
Public knowledge projects
Back in the summer John Gertner wrote a long piece for the NYT about the opportunities and threats to Wikipedia from generative AI. It’s a great article, with a lot of technical detail, including the fact that administrators have used ‘AI’ tools since at least 2002 to support the work of monitoring content, reducing vandalism and producing multiple language versions of valued pages. But while the article does a good job of balancing the technical issues, I’m mainly concerned about the risks to Wikipedia as a social project.
Like other open knowledge projects, Wikipedia and sister platforms WikiData and WikiMedia (where I source most of my images) are as biased as the human systems that support content production. For sure, contributing to open projects is a generous thing. But the people with the spare capacity to give and the confidence to think their contribution matters tend to be of certain kinds. Maari Maitreyi, a knowledge justice work with Whose Knowledge, recently reminded fans of the open commons that only 22% of the entries in WikiData concern women and only 0.3% of sources are of African origin. These are among the founding biases that have been replicated in large language models, all of which have hoovered up Wikipedia content, with its vast coverage of topics and its consistent style and metadata.
But what makes the human project different to the large language models that have scraped it for content is that humans can always work for a better version, and feel the injustice of the current one. Maitreyi:
Wikimedia Foundation has been putting considerable and admirable efforts to diversify. Among these efforts include the advent of a global Knowledge Equity Fund, which was founded after the killing of George Floyd in 2020, to commit to processes of racial equity and help offset inequity in knowledge production. And such efforts are paying off. The diversity of content is increasing slowly year by year. But these efforts are still a slow work in progress and seem to require continuous pushing by Wikimedians and knowledge justice advocates to keep the efforts kicking.
And Maitreyi has critical questions to ask the open community, including this existential one:
Is an absolute open knowledge culture really the best approach when contextualized to a world full of historical and contemporary inequities?
I can’t answer that question (though I urge you to read Maitreyi’s essay in full). But I do believe that an open knowledge culture – if it is challenged from its own margins, if it commits to putting right as many wrongs as it can – is a better place to start than a closed, proprietary model with injustice dialled in. However, this open culture, with all its flaws, may soon be overwhelmed. Or at least, the human labour that might go towards improving diversity seems likely to be spent on putting out the bin fires of bot-driven edits.
Mostly these come from human editors making use of auto-generated text, and referencing auto-generated sources. In fact this Wikipedia project page on the use of large language models makes clear that this should be avoided. This page has been a guiding light to me since the whole generative AI thing kicked off a year ago. I hope it’s also being referenced by universities:
But editors may be unwilling or unable to follow these guidelines. Writing is hard work, and the rewards of contributing to open knowledge projects like Wikipedia are diminishing. Maitreyi again:
when the unpaid labor of thousands of Wikimedians then goes to big tech companies to make a questionable profit, we must ask ourselves; did we sign up for this? Never mind the recklessness with which these corporations are imposing systems of “AI” and automation onto vulnerable populations. Why should we contribute free labor to produce open work only to have corporations subsume the work and close the knowledge gate behind them using their own shutdown copyrights?
It's worth looking at the fate of another community knowledge sharing site, Stack Overflow (though certainly more flawed than wikipedia). Use of the site fell steadily through 2023, as developers turned to OpenAI's Codex and GitHub’s CoPilot for the kind of help that they might once have found in Stack Overflow threads. The site laid off a third of its staff, and even launched its own generative interface in an attempt to stem the tide. All these generative models were trained on Stack Overflow discussions. While they have undoubtedly allowed coders to be more productive in the short term – though this is a mixed blessing for the actual coders – they are also destroying the community that produced the coding solutions in the first place. In fact, coders didn’t only use Stack Overflow for tips and tricks but to discuss a range of issues affecting their work, that may – who knows? - have given them other satisfactions. Incidentally, the same ‘state of AI’ report that finds coders turning away from Stack Overflow to personal helper-bots, also finds that they are sceptical about the claims of generative AI. Sceptical, but hooked.
The digital commons is a differentiated landscape, with different values and social models: open source is a different economy to open journalism or open science or open education. But one thing all open communities have in common is valuing community over maximising the profit from knowledge work. This makes it unlikely that open economies have much to gain from partnering with champions of copyright, however these may seem to be defending ‘content’ from the new content engines. Paul Keller of openfuture.eu argues that it is wrong-headed to look to the big publishers and their lawsuits for help:
Much of this digital commons consists of works that are free of copyright, openly licensed, or the product of online communities where copyright plays at best a marginal role in incentivising the creation of these works… the response to the appropriation of these digital commons cannot be based on copyright licensing, as this would unfairly redistribute the surplus to professional creators who are part of collective management entities.
The failure of the EU AI Act to protect open source development and the emerging open model ecosystem – under pressure from big corporate developers in the EU - seems likely to undermine what Paul Keller argues are ‘the building blocks of transparent and trustworthy AI’: open models, in partnership with open content communities. Unless public bodies and universities work together to make such developments more possible, only the wealthier organisations will be able to achieve the necessary closures and enclosures, attract the necessary knowledge-producers and afford the services that research and teaching require. Only the very wealthiest will be able to develop or buy into safe and ethical models that meet the needs of their academic communities.
Random and improbable events
Generative AI has become its own self-fulfilling prophecy. Too many big players have bought in, built on and bigged up generative AI for the hype to easily be put into reverse. But in the real world, beyond probabilistic modelling, improbable things happen. And it is – just – possible that the bubble may burst.
The tech corporations that are carrying the eye-watering, reservoir-draining, climate-heating costs of development have to find a way of making it all pay. There are clouds on the profitability horizon, such as the unknown costs of litigation over copyright and privacy violations, and those negotiations with content providers. While some users may be willing to overlook racial and gender bias for the sake of easy copy, they may be less forgiving of non-consensual AI porn and child abuse images. In 2024, almost half the world’s population goes to the polls. Many will be carried there on a tide of synthetic disinformation. Bad outcomes will not easily be forgotten.
More prosaically, the exponential improvements in performance that were promised earlier this year not been realised – in fact, many models seem to be getting worse. Is it model collapse, or (as the bloggers of AI snake oil suggest) cost-cutting behind the scenes, or, worst of all perhaps, people just starting to realise it’s a bit rubbish? It doesn’t help that every attempt to improve things has unpredictable effects on model behaviour, which makes life unpredictable for anyone trying to build apps, workflows and APIs on top.
If by one or other of these random chances the bubble should burst, that would not be good news for any academic projects that have come to depend on generative AI models or interfaces. At the very least, a lot of opportunities would have been lost, including the opportunity to plan for some other things while ‘generative AI’ was filling the future horizon. Climate collapse, for example, or galloping inequity, or the rise of authoritarianism and unreason in a world at war. The chances are against it, I know. But in a sector that is supposed to think critically and widely, it should be possible to hold this improbable outcome in mind as well as the widely expected ones.
On the subject of thinking differently, my wonderful friends and colleagues, Laura Czerniewicz and Catherine Cronin, recently published a book called Higher Education for Good. While it documents some of HE’s failures, particularly in the early chapters, it also brings together a wealth of hopeful responses and adaptations, particularly from universities in the global south. And improbably, against all the logics I just outlined, the book is freely and openly available. I recommend it as a source of hope.
Lots to think about, Helen, from lots of angles,. Thank you so much for illuminating some dark corners.
What a tour de force. <3