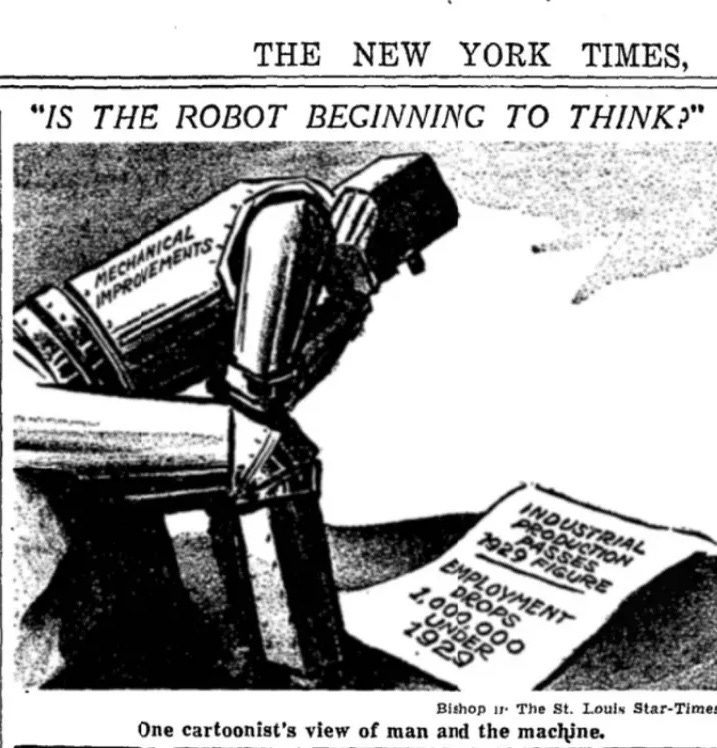
In my piece on risks to students from generative AI, I left a couple of ideas to explore in more detail. One was about the risks to critical thinking, and the other was this one, about the risk to students as future workers.
What is the likely impact of generative AI on the graduate job market? According to the consultancies and think tanks, it’s everything, everywhere, all at once – and education had better run to keep up. The world is certainly changed, but for the moment at least this has nothing to do with the value of large language models to address economic and social challenges, and everything to do with their marketisation.
Big tech companies are also venture capitalists. Google has GV, Microsoft has M12, Salesforce has Salesforce Venture, even OpenAI has its own venture fund that announced its first investments (in specialist use-case start-ups) just two weeks after launching ChatGPT. Venture capital thrives on potential, especially the potential to capture a new market. Use cases are for the little people. In fact, the initial release notes for GPT-4 include just one example of use (a short socratic dialogue) and no further ideas for application:
So it’s over to the ‘community’. And universities are stepping up to the challenge, providing use cases directly in new learning, teaching and assessment practices, and indirectly by preparing students to work ‘alongside AI’.
Remember that as soon as the foundational models were launched, their developers immediately tried to put the brakes on, announcing that what they had created was in fact a ‘giant AI experiment’ that needed regulation rather than a product designed to meet an established need. Language models were integrated into platforms and enterprise systems – into plagiarism detection and search engines, for example, two key platforms for student learning – without any evidence that users would benefit, only boardroom negotiations about slices of pie.
I’m not suggesting that this technology, or any technology, is ‘only hype’. Hype is as much a part of the new technosocial structure as the nvidia chips it runs on. Use the language of learning to describe computational functions (‘learning’, ‘intelligence’, ‘knowledge’, ‘mind’) – or the language of computation to describe human learning - and computational models will find educational uses, more readily at least. Describe professional work as ‘human / AI collaboration’ or ‘humans in the loop’ of computational systems, and different jobs will be specified for graduates to do. Prompt engineer? AI fact checker? Empathy analyst? These are not realities until we name them.
Work inside the engine
The positive version of the ‘AI’ future is that artificial ‘intelligences’ will free human beings to do ‘human jobs’ by taking over the tedious, repetitive and uncreative tasks. Notice that already this configures technologies as task-doers, rather than at tools for workers to use. We’ve seen that the language model industry is producing new kinds of work and new working relations, and that they are not especially creative or liberating ones. I checked out the jobs available through a typical data labelling platform (the ‘middle layer’ of labour I wrote about in that earlier post) and found the work described very differently to data customers and to data workers. On its pages for ‘AI innovators’, the platform boasts of having delivered more than 40 million hours of data annotation and labelling (‘luckily we love tedious work’).
Meanwhile the ‘scalable data labelling workforce’ doing this tedious work are recruited in Kenya, Nepal, Venezuela, and other low to medium waged economies, with the promise of ‘meaningful work’ that will ‘contribute to your community’. In these locations, the offer of reasonable piece rates and ‘foundational digital skills [for] high tech workplaces’ (a two-week training programme) may be worth the lack of security. But the labour inequities are hard coded into the business model, and clearly colonial.
Some UK graduates may be among the ‘AI innovators’ who are buying in data work. That is the UK government’s vision for higher education, but the truth is that the UK is carrying some significant disadvantages in becoming an AI launchpad, and the number of graduates in exciting development roles will be relatively small. Some will be employed in middle management roles, managing the people and work flows that make up the data ‘engine’. But many will find themselves inside the engine: the globally distributed, plug-and-play, tedium-loving workforce in the cloud, expected by some to be worth $14 billion by 2030 and actively targeting graduate labour.
Screenshots from a different data labelling company, Scale.ai show how the services provided by the ‘GenerativeAI Data Engine’ might require graduate level skills. Labelling, classification, ranking, rating and comparison, as well as transcription, translation, ‘generation’ and model evaluation – these cover Bloom’s taxonomy of learning outcomes very nicely, and are no doubt already being repackaged (perhaps via text generation) into the ‘AI curriculum’ the future needs.
For all they skills they bring to the AI enterprise, data workers are precariously employed and relatively disempowered, constantly monitored, losing pay if their accuracy falls, and sometimes dealing with distressing images and text. They are on the wrong side of what Shoshana Zuboff in Surveillance Capitalism calls the ‘division of learning’ between those with agency in the data economy, and those without. Those with ‘meaningful work’ and those whose work is so minutely disaggregated that they are prevented from seeing what meaning, if any, it might have. The exploitative nature of crowd work is explored in Ghost Work (Gray and Suri 2019), and regularly reported on by Karen Hao in MIT Technology Review. Rather than building a smooth pipeline into this work, an ‘AI ready’ curriculum could be awakening students to its inequities and risks.
Work in the mainstream
Companies directly involved in generative AI are a small part of the graduate job market. But they are a powerful signal to other corporations of how work can be reshaped. Their products are being embedded into other organisations directly through the development of bespoke models and services, and indirectly through productivity platforms (Microsoft, Google, enterprise systems). As I have argued, this is the real business case for generative AI, and these are its real customers.
Is graduate work likely to become more creative and fulfilling as a result? Productivity can feel good when it first arrives. We work faster, the toys are fresh out of the box and nobody wants to be last to play. But the companies buying the productivity gains can only realise them if they are prepared to extract more work, employ fewer people, and/or make them more precarious. Otherwise skilled workers being more productive can demand higher wages.
Tech-driven productivity always means restructuring the work people do - standardising work flows, and splitting work into less skilful components. This is often termed ‘digital taylorism’ to reflect its origins in the ‘scientific management’ of factory labour, pioneered by Frederick Winslow Taylor in the 1880s. But a better term might be ‘Babbageism’. As well as devising the first model for a working computer – in fact, as the main reason for devoting himself to this eccentric pursuit - Charles Babbage was a pioneer of labour division, control, rationalisation and surveillance. A full fifty years before Taylor, he documented his methods in ‘On the Economy of Machinery and Manufactures’. Meredith Whittaker, in a brilliant re-reading of Babbage, records his insight that:
dividing a complex task into simpler component parts, and designating these simpler parts “low skilled,” could justify paying the people who perform each part less. At the heart of the Babbage principle sits the implied right of an employer to define the value of work and the worker… pegged to classifications of “skill,” which was assessed based on the scope of the task performed.
I feel sure the substack will feature more Babbageism in the near future. But here let’s pause for a moment to consider what it means for educators to restructure the university curriculum around the new classification of ‘graduate skill’, based on the functionality of today’s computers. A recent study of professional work in Europe, 1995-2015 (published 2023) found that:
‘computers mostly replace routine tasks… But the results presented in this article also show an even more significant increase, over the same period, in the reported levels of repetitiveness and standardization of work processes (and thus in the levels of routine).’
So, perhaps counter-intuitively, automating routine tasks results in more routine work being done, just as Babbage foresaw. The fear of ‘losing your job to AI’ is a powerful narrative that may push workers to accept restructuring, even if full automation remains a mirage. Mike Cole of the Oxford Internet Institute gave evidence about these effects to the Commons Select Committee on ‘Connected Tech’ a few weeks ago:
With each introduction of new technology there is a degree of deskilling that is necessary as you break up the complex tasks into simpler ones so that computers or machines can perform them. Yes, we have seen a polarisation of skill. There has been wage growth and an increase in some higher-level managerial positions but also a large increase in lower wage and deskilling of positions.
So technologies like GenAI are contributing to the falling incomes of relatively skilled people in developed economies as well as hyper-exploitation in less developed ones. Graduates may be able to do tedious work more quickly, but can only replace it with more meaningful work if their employers value that work and value graduates who can do it. Perhaps graduates can improve your income by doing more than one ‘tedious’ job at a time - if they are enterprising and energetic enough, as these ‘overemployed hustlers’ reported to Vice back in April. But working two jobs, or three, or five, is not for everyone (obviously), and it certainly doesn’t increase the meaningful work available overall.
What is it good for?
Gary Marcus is one of the most critical commentators on generative AI and its impacts. A recent post from his ‘Road to AI we can Trust’ substack suggested that only ‘spammers, scammers and shammers’ are finding good uses for ChatGPT, though earlier he conceded that coders and undergraduates are also fairly satisfied users. Coders because:
its autocomplete nature is fabulous for their line of work, and they have the training to detect and fix the not-infrequent errors,
and undergraduates because… well, auto-complete your own reasons here.
Marcus may be an outlier in believing that the GenAI bubble is about to burst, but it’s clear that after half a year of public access and publicity, most business users are not convinced of the benefits. A survey by the Pew Research Centre in May found that only 14% of US adults had tried ChatGPT and of those, only a third found it extremely or very useful. The foundational models seem to be getting worse (more inaccurate, unstable) rather than better. Businesses are banning their use by staff, because of security risks and the unreliability of their outcomes, while still integrating generative capabilities into enterprise systems and bespoke models because, guys, we need those use cases!
So what is generative AI actually good for, that would justify a belief that it is going to transform the graduate workplace? The primary use case – confirmed by an article in the Journal of Business strategy (June 2023) and by the latest McKinsey report (June 2023) – is content production, especially for sales and marketing, social media, and internal communications. Think of words that nobody needs to read and you have a use case. A legion of web sites, apps and experts have sprung up to help businesses turn on their own copy-hose. For example, among the top search engine hits for AI content generation is this advice from a company called Zapier:
After three years of managing our community, it's sometimes difficult to think of new ideas. But ChatGPT helps me come up with topics for our daily watercooler threads and LinkedIn polls.
Zapier also recommends GPT-4 for ‘rapid research’. A happy user reports that:
We collect 90% of our facts and data from GPT-4, but we don't use it to find sources… as most of the links the tool returns don't exist or are out of date.
Then there are all the infographics and infomercials on using GenAI for search engine optimisation. Training a large language model on clickbait, then scraping the universe of clickbait for more training data, turns out to be great for producing clickbait but – and I think you knew this was coming –
Common pitfalls of such content are factual inaccuracy, unhelpfulness, or unoriginality, which can lead to a breach of trust, negative customer experiences, and reduced engagement.
With auto-generated content flooding every communication channel, engagement is bound to be a problem. There may be more emails, product reports, social media posts and watercooler threads - a recent job ad called for a copy editor to produce 250 articles a week - but no-one has time to read it, so they turn to GenAI to search, summarise and prioritise it for them. The ‘content’ mill, already a howl-around of disinformation, non-information and data, is being geared even further towards attention deficit. And this is the primary way that work will be revolutionised.
As well as content at scale, though, there is another group of use cases: providing a natural language interface for technical tasks such as data analysis, data management and coding. This is intuitively more hopeful. Tools like GitHub’s Copilot - based originally on OpenAI’s Codex language model, and more recently on GPT3.5 - have been greasing the wheels of programming for a couple of years now.
Professionals have a good understanding of the pitfalls. One typical developer recorded his frustrations with Copilot, wondering ‘if the next twenty years of programming might be undoing AI-generated code’, but still felt unable to give up on the feeling that ‘programming is now a game’, a dialogue rather than a one-way slog. This is something I have heard from amateur coders, academic computer scientists and expert developers alike. Also, perhaps because of the sheer volume of MicroSoft-related ‘how to’ content sucked up in its training, ChatGPT seems pretty good at helping spreadsheet jockeys write macros and generate charts to order.
But developers are increasingly fed up with the many ways CoPilot gets things wrong, and with explaining to clients that even when it gets things it gets right, human developers are still needed for overall design, purpose and context. For ‘meaningfulness’. Just as there are copyright battles in other creative industries, there are challenges to the way coding models are parasitic on the historic content of Stack Overflow and GitHub and developer Reddits. Making technical work more accessible – or apparently more accessible – to less skilled and experienced workers means that faulty and vulnerable code is being produced and propagated. And just like creatives in the text, image and music industries, developers worry about the future. According to one insider:
For the industry, it’s really bad because people aren’t learning to actually code. They don’t understand how it works.
Workers without this understanding might still get employed because they are cheap. Or, more likely perhaps professionals with expertise from the before times will work faster and shut out the novices. Whether either of these scenarios will lead to higher wages and more meaningful work overall seems doubtful.
Beyond the current horizon – artificial general intelligence
But perhaps the two use cases I have identified for GenAI - generating fluent bullshit and providing a natural language interface for technical work - are only the earliest examples of what ‘artificial intelligence’ can do. Large language models have already been hailed as general ‘reasoning engines’, for example in this excited paper from Microsoft Research. More commonly, the cognoscenti tell us that ‘artificial general intelligence’ is not here yet, but only a few years away. The term ‘general’ begs as many questions as ‘artificial’ and ‘intelligence’ do. Until recently, ‘AI’ itself was the chimera that was just over the horizon. If AI is now real, and here, and worth the cost of your subscription, something else must be just over the horizon. Something to explain why the AI we have falls short of what was promised. Something that can use that shortfall to generate more expectation and investment. AGI is that thing.
Sam Altman’s latest definition of AGI is simply: ‘AI systems that are generally smarter than humans’ (my italics). In 2018, he offered more detail:
highly autonomous systems that outperform humans at most economically valuable work
Personally, I think the first two parts of his definition – performance plus autonomy – will be difficult to achieve in the same system without some paradigm shift in computation. But even if autonomous, highly performing and widely applicable systems were possible, I think they would fail Altman’s third test of economic value. Why do I think so? First, by analogy with robotics, second with a bit of theory, and third by looking at the actual trajectory of ‘AI’ at work.
Robot parcour
We have since the industrial revolution (at least) had machines that could outperform human beings on physical tasks of many kinds: weaving cloth, shaping metal, digging furrows. Contemporary robots can pack boxes and bolt precision-engineered parts into cars and aeroplanes. These robots are narrowly specified, and require specially-designed settings (factories, production lines) with associated routines. The tighter the specification, the better the performance.
There are certainly projects to develop general or humanoid robots, able to perform a wide range of tasks with human or more-than-human precision. Meet Ken, for example, the blue-eyed boy (literally, I’m afraid) of ENCS Humanoid Robotics Project. While Ken is only experimental at the moment, Atlas, named (obviously) for his strength, can do parcour when programmed with the right moves in a special lab environment. The economic value of robot parcour is not high, but the show-off value to developers Boston Dynamics (who actually sell ‘mobile industrial robots’) is presumably worth the expense. The Atlas robots are even programmed to do ‘show off’ gestures after their parcour stunts to make the point.
There are also female-gendered humanoid robots with names like Erica, Gina, Jia Jia and Sophia (based on Audrey Hepburn, and not at all creepily, the wife of Hanson Robotics CEO). These ‘gynoids’ are valued for their realistic hair and skin, facial expressions, and tasks such as nursing care. They can achieve ‘full functionality’ in ways that androids cannot, perhaps because men are supposed to master actual skills like DIY and barbecuing and parcour, rather than just looking the part.
The ways these humanoid robots are gendered and racialised (and sexualised) is laughable. It is also deeply serious, and for serious discussion I recommend this preprint: When Humanising AI Dehumanises women, and Ruha Benjamin’s 2019 book Race After Technology (the link is to a detailed review). The real function of these humanoid robots is not the tasks they perform but the narratives they produce, particular narratives about the prestige of their developers, and general narratives about what constitutes humanity and (therefore) human work. There may be some spin-off benefits to real engineering projects, because as we have seen, money follows hype, and there is nothing like a bit of promotional parcour or a female robot making eyes at her (male) maker to whip up a round of capital funding. But functionally, humanoid robots are gimmicks.
Why would an ‘artificial general intelligence’ be anything more? Given the huge costs of building generality - the scale of development required, the evaluation and testing, the weakening of specific capabilities - any benefits could be realised at a fraction of the cost by giving human workers more specialised algorithmic tools. And even if a generalised ‘artificial intelligence’ could be found useful work to do, its development would have required such an immense investment of funding that any uses would emerge only in the shadow and service of its own hype.
Finding the ‘g’ factor
An argument against the analogy with robotics might go like this: ‘intelligence’ is different to the capacity to perform physical tasks, because physical tasks are endlessly various, while intelligence is a singular, generic ability from which derive all the cognitive capacities that humans, animals and computers have. As Sam Altman puts it, ‘the first AGI will be just a point along the continuum of intelligence’. A point that will continually advance, like computer power according to Moore’s law, so that just by improving along this one dimension of thinking power, AGI will soon be able to outperform human minds on mental tasks of all kinds.
This ‘general’ dimension of intelligence is known in psychology as Spearman’s ‘g’ factor. But Spearman’s ‘g’ factor was contentious from the start (“Professor Spearman has drawn over-hasty conclusions”, Michell 2023). The link is to a paper in Theory and Psychology that reports and re-evaluates contemporary (early twentieth century) objections to Spearman’s conclusion - that a single, quantifiable factor lies behind observable differences in mental performance. It shows how the statistical tests that Spearman’s name is most associated with - ranking and factor analysis - were intrinsically biased towards reaching this conclusion. And it records some of the consequences of his flawed assumptions: eugenics as well as psychometric testing are built on Spearman’s quantitative approach to intelligence.
Bad outcomes do not necessarily prove bad science. But in maths and computation, ‘general’ computational intelligence has also been contentious. This preprint from cognitive scientist Iris van Rooji and colleagues works from first principles to show that:
creating systems with human(-like or -level) cognition is intrinsically computationally intractable. This means that any factual AI systems created in the short-run are at best decoys.
This stuff is hard compared with poking fun at gynoids, and I will try to do it more justice in another post. But I was excited to discover that there are AGI doubters in cognitive psychology and computation as well as in critical technology studies where you would expect to find them (and where I feel more at home).
AI at work
The early twentieth century entanglement of statistics, ‘intelligence’ and race/gender politics is highly relevant to present day ‘AI’, as I explore in other posts. In this discussion of AI and working futures, my main point is that working ‘AI’ systems tend not to be general, but narrowly specified to the task or problem space.
It took twelve years to develop Deep Blue from first prototype to beating world champion Gary Kasparov at chess (in 1997) and it was another 20 years before AlphaGo beat world champion Ke Jie at Go. On both occasions I remember hearing that human beings should pack up our smarts and go home. Machines had beaten us into second place. But these projects were the cognitive equivalents of robot parcour, effective in a rules-based, bounded and mathematically-defined problem space, with sequential moves and clear rules for success. As with industrial robotics, there were spin-offs from these headline-grabbing projects, including some of the computational developments that have gone into genAI, and a some of the venture capital. But they were not themselves economically viable: they were demonstrators.
Most real world applications of what is called ‘AI’ follow the same rule of narrow specification leading to speed and accuracy. Advanced algorithms can help to identify potential new antibiotics from drugs databases, find cancer signals in medical images, and predict protein structures from their chemical composition. These algorithms are part of a highly specialised workflow that includes human scientists and technicians, lab settings, and a host of other tools such as x-rays and MRI scanners, gene coders, electron microscopes and automated pipettes for producing crystalised proteins. Many of these algorithms are so specialised they can be used only with a specific database, or with a particular brand of medical scanner.
Generative AI performs over much broader problem spaces - language, images, music, code - and is probabilistic rather than deterministic in its pattern finding. As a demonstrator, it has been effective beyond the dreams of its makers. As a professional tool, it has many limitations: it is just not accurate enough for use in healthcare, which is where more conventional ‘AI’ applications have been successful. As a driver of economic value, it is struggling to prove itself. But as a precursor to some more ‘general’ intelligence? The direction of travel has been entirely the other way. Ongoing human interventions are needed in the middle layer (data enrichment, reinforcement learning) and at the user interface (prompt engineering, fact checking) to adjust the outputs to the ‘rules’ as human beings understand them. Businesses are not looking to the foundational models but to more bespoke and specialised ones to meet their needs - domain specific and even hyperlocal models trained on very specific data to achieve specific tasks. So to the extent that their capabilities are more general than playing Go or finding traces of lung cancer, generative AIs are also less accurate, less reliable, more fragile, and more dependent on human supervision.
All this suggests that ‘intelligence’ – if we insist on using the same word for machines, humans and other species– is multiple, diverse, and modular, rather than single, ‘general’, and integrated. The ‘G’ in the middle of ‘AGI’ names something incredible about minds as we know them, but completely fails to explain how the experiments with algorithms we have made already are going to address its challenges. Even if you are philosophically inclined to find ‘mind’ in any complex enough system, predictions of an artificial ‘general’ intelligence in the workplace do not hold up to economic scrutiny. They do speak to certain theories about human intelligence that have been around for well over 100 years, and that have consequences we would do well to examine through a historical lens, as well as a psychological one, before including them in our narratives about working futures.
Meaningful work
We can agree, I hope, that the future of human work should not be defined by the latest tech hype. An ‘AI curriculum’ of prompt engineering and fact-checking should not be the limit of graduate horizons. But what about more thoughtful contributions on the development of ‘soft’ skills and ‘human’ capabilities that will be of lasting value, whatever the next disruption from silicon valley? There is a nice example of this genre here from Mark Rollins (these are the first of twelve points).
I also agree with many of Rollins’ solutions: project-based learning, interdisciplinary work, formative and performative assessments, peer and self-reflection, and learning portfolios. I agree that it would be wonderful for human development to take centre stage in curriculum design - perhaps humanities departments might even get a reprieve as a consequence. Still, I worry when ‘human’ capacities are positioned against technology, valued for being unlike something defined as capability, just not fully human. I think this repeats the error of ascribing intellectual capabilities to algorithms, rather than seeing them as tools for intellectual work. Human beings also invented the tools of mathematics, logic and computation. Data is also human work.
Whatever we take ‘humanity’ to mean - and there will be many as many definitions as there are human cultures and identities within them - the use of tools and technologies seems to be involved. So the alienation of people from technologies may be more about how technologies are owned and organised and less about the need to preserve an unchanging human essence against them. Denouncing big tech from the perspective of humanism, or a particular romantic kind of humanism, can mean harking back to fixed ideas about what human nature is, and therefore what different kinds of human being are good for.
So perhaps Rollins’ second point could be expanded to include critical thinking about the whole premise of ‘artificial intelligence’ and the idea that whoever gets to define it also gets to define what ‘human’ beings and capabilities are as well. I have my own thoughts on this: a post on the history of ‘artificial intelligence’ as a term, as well as posts on critical thinking, on thinking and language, and more. But these are just my thoughts. What matters is that the conversation should happen, in and around the curriculum, with teachers and learners involved.
Conversations might start with questions about critical thinking, creativity and problem solving in different subjects of study. What do these look like when people are practicing them (experts and non-experts)? What value do they have? What computer-based tools are relevant, and how do they change the practices involved? At what point, if ever, would it be helpful to see the algorithms themselves as critical, reasoning, creative, intelligent? What would be lost and gained in taking this perspective? How does this subject area (with all its resources of theory, method and practice) understand ‘learning’? What do other disciplines say about learning that offers a different point of view?
My hope is that, rather than a curriculum ‘for AI’, these conversations would create space for learning that addresses human challenges. Getting life on earth out of the mess that fossil fuels and rampant production have made of it will take all the graduate labour we can produce and more. Nobody is going to be without meaningful work - not climate scientists or green energy specialists or engineers or geologists or computer scientists or materials chemists or statisticians. Not a single person educated in the STEM subjects beloved of governments everywhere can be left idle. But nor are we getting out of this without social scientists to help us weather the social and economic and political storms, humanities graduates to develop new laws and policies, new philosophies and imagined futures, and professionals committed to a just transition in their own spheres of work. And there are other crises, entwined with the climate crisis, that graduates need and want to address, such as galloping economic inequality, crises of democracy and human rights, food and water shortages, and the crisis of care. Universities can offer fewer and fewer guarantees of secure employment and decent pay, but they can offer meaningful work, justifying students’ investment in the future.
The longer you look at the things ChatGPT can do, the more they resemble what David Graeber described as Bullshit Jobs - jobs that don’t need doing. While I don’t agree with the way he singles out specific job roles, Graeber is surely right that more and more work involves doing things with data and information and ‘content’ that has no value beyond maintaining those systems. And one claim he made that is borne out by workplace research is that meaningless work is bad for people’s mental health.
It’s a nice little aphorism that ‘if AI can do your job, AI should do your job’. But here’s a different one. If AI can ‘do’ your job, you deserve a better job. And if meaningless jobs are bad for workers’ mental health, how much worse are they for all our futures? The phrase ‘fiddling while Rome burns’ hardly begins to cover our present situation. As the polycrisis heats up, the crisis of not enough water-cooler text is not something any graduate should have to care about, nor any university curriculum either.