AI literacy and Schrödinger's ethics
Reviewing the Russell Group's Principles for the Use of AI in Education
This week the Russell Group of 24 UK universities published some shared ‘Principles on the use of AI in education’. No doubt they will be consulted by many other organisations looking for guidance. RG universities are among the most powerful institutions anywhere in the world when it comes to shaping knowledge practices and culture. So they can be reasonably confident that what they have to say on this subject will be heard.
The Principles deal only with use by students and teaching staff, with a focus on academic integrity. Of course, universities may have other principles in discussion, about protecting academic jobs and conditions for example. They may have principles about using (or not using) GenAI in admissions, grading, academic integrity boards, for identifying students ‘at risk’ and in other decisions critical to students’ outcomes. They may have principles about reviewing the safety of their platforms and systems when AI functionality is embedded into them. There is a precedent for this: in April when Turnitin suddenly included a pilot GPT ‘detection’ service with its standard offer to universities, UCISA negotiated an opt-out and many universities took it. Universities may decide on principle to fund and develop ethical alternatives to mainstream GenAI models – for example if they decide that these models through inequity, bias, lack of transparency, violations of copyright, carbon footprint etc etc are incompatible with other values universities hold dear.
As I say, these are principles that may be under discussion within the Russell Group, and there may be good reasons for talking about them less in public than the academic integrity issue. Student use of GenAI to help with assignments is widespread, perhaps close to 100% if conversations with students and young people are anything to go by. It’s a gift to anyone who wants to paint universities as irrelevant, or morally compromised (because they are allowing students to ‘cheat’, or failing to stop them ‘cheating’) or just confused and weak. So it is important to have a collective response on this issue, and the Principles offer that.
This is what they say:
1. Universities will support students and staff to become AI-literate.
2. Staff should be equipped to support students to use generative AI tools effectively and appropriately in their learning experience.
3. Universities will adapt teaching and assessment to incorporate the ethical use of generative AI and support equal access.
4. Universities will ensure academic rigour and integrity is upheld.
5. Universities will work collaboratively to share best practice as the technology and its application in education evolves.
I would summarise this as: (Principles 1 and 2) teaching staff and students must decide what constitutes ‘effective’, ‘appropriate’ and ‘ethical’ use of generative AI, using their skill and judgement, and (Principles 3 and 4) curriculum teams must ‘adapt’ teaching and assessment to include GenAI, while ‘rigour and integrity’ continue to mean exactly the same.
I’m right here for critical digital literacy – it’s what I research and it’s why I started this substack - and for trusting staff and students to lead the conversation. I could not agree more with ‘empowering’ academics to make decisions that work for their students, in their course and context. But knowledge is not empowerment without the power to act, and resources to act with.
The Principles list a range of known harms: harms to user privacy, to intellectual property rights, to people discriminated against and exploited by GenAI production, and the risks of what is termed the ‘inaccuracy’ of GenAI outputs. But they don’t say how knowledge of these harms should inform choices around use. Given these harms, how can individuals determine what is ‘ethical’? How do curriculum teams decide what is ‘appropriate’, in the absence of any research into how these harms play out long term, or for particular students, or in relation to particular tasks? Most importantly, what support is there for university members who make a ‘wrong’ judgement, as students may do in relation to their assessments, or who call out some use of GenAI they consider unethical and inappropriate, as critics of Proctorio did in relation to proctored examinations, and were sued by the platform provider? Because that is surely the acid test of a ‘principle’: is it clear what you should do? And who has your back when you do it?
In the face of these immensely difficult and complex decisions, the content of any guidance is left to Russell Group members:
All 24 Russell Group universities have reviewed their academic conduct policies and guidance to reflect the emergence of generative AI. These policies make it clear to students and staff where the use generative AI is inappropriate, and are intended to support them in making informed decisions and to empower them to use these tools appropriately and acknowledge their use where necessary.
This is where an example or two would be helpful. In their absence, I visited the web sites of prominent RG universities. On some there was essentially placeholder text reiterating the Principle that ‘guidance will be provided’. But others gave examples of use that may be legitimate from an academic conduct perspective. From Nottingham: ‘(for instance, to research a topic or formulate an essay structure)’. From Birkbeck: ‘helping you summarise and analyse complex materials; extract key findings; break writers’ block; and highlight grammatical errors’. And there are some exemplary student-facing policies, given the uncertainties of the situation, such as this from the University of Edinburgh. It is clear, calm, reasonable, and links the harms and limitations of GenAI directly to the university’s policies on use.
Support for making ‘informed decisions’ is clearly evolving fast in some places. But it is still evolving. Meanwhile the consequences for students of ‘inappropriate’ use remain potentially life changing. The responsibility for determining and supporting ‘appropriate’ use falls with a heavy load on teaching staff. And the onus is still on individuals to make and adjudicate these decisions in a rapidly changing moral universe, one where the impacts are acknowledged to be serious, and little known.
The quantum code
Like other AI policies, the Principles rely on ‘ethical use’ to do the moral work. Over here it is helping staff to write assessments. Over there it is not helping students to write assessments. Somewhere else it is invoked in the guise of equal access (equal access to a technology that is acknowledged to be unequal in its consequences). But while it is summoned everywhere, ‘ethical’ is nowhere defined. Harms are indicated, but it is up to the ‘AI literate’ individual to decide when these harms matter enough for use to become ‘unethical’ or ‘inappropriate’. The only ‘ethical codes’ referred to in the Principles, besides the academic integrity policies I went looking for, are codes provided by the AI industry itself. We are warned that:
‘while ethics codes exist, they may not be embedded within all generative AI tools and their incorporation, or otherwise, may not be something that users can easily verify.’
A kind of Schroedinger’s ethics then. What an AI-industry code might say if it could be found alive, whether it is likely to accord with the ethics of the university, how university members could judge that, who would be responsible for any violations, and how this quantum code would offer any mitigation of harm… these questions are unfathomable. And it is never clear that the harms concern higher education in a particular way or that higher education has a particular duty to respond, except perhaps in relation to academic integrity. In post on the potential risks to students, to teaching staff, and to knowledge economies, I lay out why I think the responsibilities go much further.
Now you see it, now you don’t
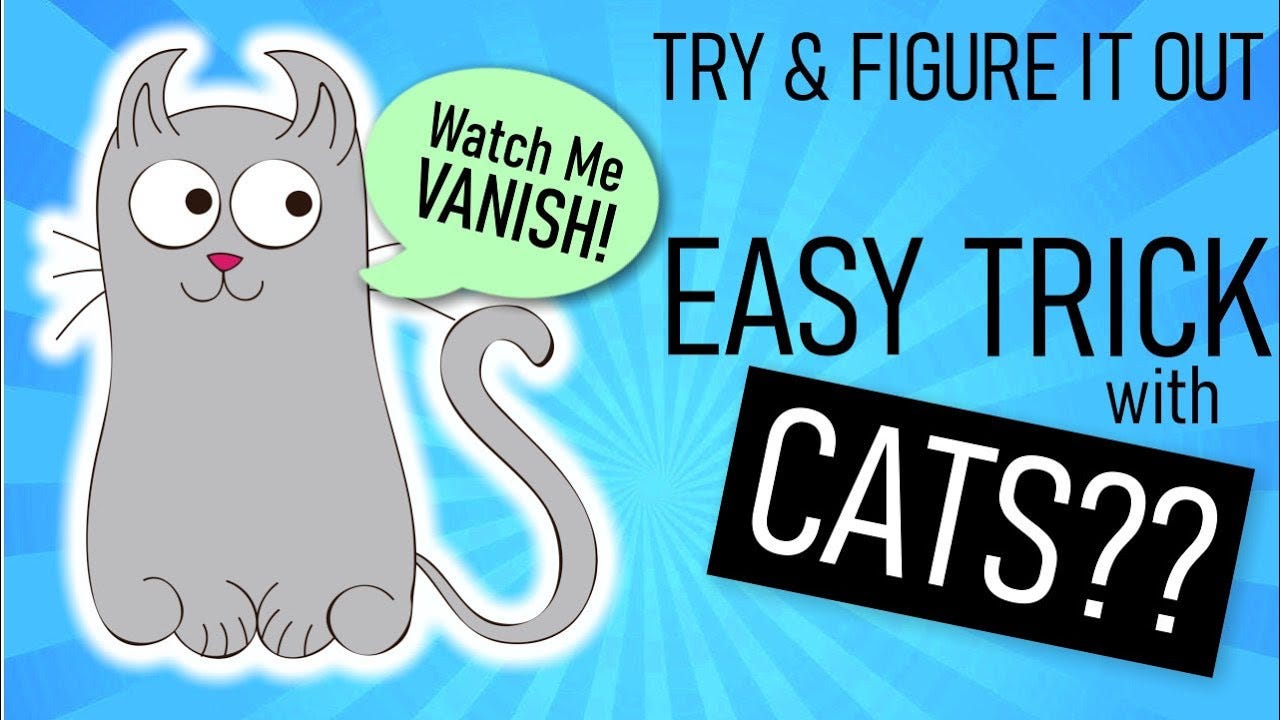
But we do have some idea what a code of ethical practice could look like, and specific to the education sector. The original draft of the EU’s regulation on AI, released in 2021, defined uses of AI in education ‘high risk’. Specifically:
AI systems used in education or vocational training, notably for determining access or assigning persons to educational and vocational training institutions or to evaluate persons on tests as part of or as a precondition for their education should be considered high-risk, since they may determine the educational and professional course of a person’s life and therefore affect their ability to secure their livelihood. When improperly designed and used, such systems may violate the right to education and training as well as the right not to be discriminated against and perpetuate historical patterns of discrimination.
As such, these uses would be subject to a wide range of requirements including: risk management systems, data governance, technical documentation, accuracy, robustness, cybersecurity, detailed record keeping, transparency to end users, and human oversight (both built in to the system and monitored by the system user: ‘Humans should be able to intervene and stop the system’). None of the proposed uses of GenAI in assessment that I have read would meet these requirements. An accompanying Human Rights recommendation, directed by the Council of Europe to ‘anyone who significantly influences – directly or indirectly – the development, implementation or effects of an AI system’(including universities) states unequivocally:
‘DO NOT use AI systems that are complex to a degree that they cannot be subjected to human review and scrutiny by appropriate standards of transparency and accountability.’
Before being finalised this year as the Artificial Intelligence Act 2023, all these provisions had been disappeared or watered down. The Act in its entirety was made voluntary on organisations rather than binding by law on member states. Nobody doubts or denies that this was because of hefty lobbying by the AI industry (see this article by Anne Gerdes and for a less academic take this ‘exclusive’ from Time Magazine). There were also interventions by the US government, alongside the UK, Canadian and Israeli governments to exclude commercial businesses and state security apparatus. This should tell universities what happens when codes of practice are developed in ‘partnership’ with deeply invested interests.
So what?
Even the draft code fell short of what was needed, in the opinion of most human rights experts. And yet, while the Council of Europe still seemed willing to put a brake on AI deployment, a useful body of research and policy was initiated, such as this paper on AI ethics, written by a team in UK HE, that looked at diverse codes and how they might mitigate some high level risks. If the university sector wanted to understand the risks more deeply and particularly, and take a collective position on mitigation, it could be building on these resources. It could be defining its own code of AI ethics, with its own values and stakeholders, independently of the AI industry and whatever it may or may not ‘embed’ into its platforms. Without collective action of the kind it (briefly) took with Turnitin, universities will have little choice but to adopt the latest technical ‘fixes’ from the very industry that is creating the problems. ‘Ethical AI’ is already an industry within an industry, much of it run from departments within existing AI mega-corporations, set up to disarm external regulation and/or reassure consumers. These departments, notoriously, are stood down if they get too expensive or too uppity to meet the brief.
Universities do not have the excuse that the technology is beyond our understanding. Until very recently, the developments that power GenAI were being made inside, or at least in partnership with, university research labs (see for example Meredith Whittaker’s unwavering essay The Steep Cost of Capture on how this situation was overturned, and big corporations took the lead). Higher education is one of the few sectors that could bring together the expertise and funding to produce more ethical alternatives to existing models. Universities have a recent history of tough conversations around #MeToo, decolonisation and freedom of speech, and could be hosting genuinely critical conversations about AI futures. Drawing on expertise not only in computing and data science but in social science and critical technology studies. Refusing the narrative of inevitable ‘disruption’ to the futures of graduate work. Daring to be led by students, in spaces where they can be honest about the extent of their use, their AI dreams and fears. Working out how to centre the people and communities who are most likely to feel the negative effects.
I know these conversations are happening in some parts of some universities. The Principles may help by clearing ground over in the academic integrity corner so that the focus can move to these wider intellectual and moral concerns. But they miss an opportunity to open up and support those conversations, by not providing a sense of the particular jeopardy universities face in relation to the harms they name. Principle 5 could be extended from ‘sharing best practice’ to something much more concrete: a code of ethics and practice that stands independent of the AI industry and is robust enough to deal with its disruptive power.
Absolutely spot on. I started to read the Russell Group principles during the third round of revisions on my Blueprint for an AI Bill of Rights for Education, and thought “oh, someone’s beat me to it.” And then about halfway through I was scratching my head. This is one of the best analyses I’ve seen of this document; thank you for this. Looking forward to reading more of your work!