Labour in the middle layer
It's becoming clear just how much human work maintains the illusion of intelligence
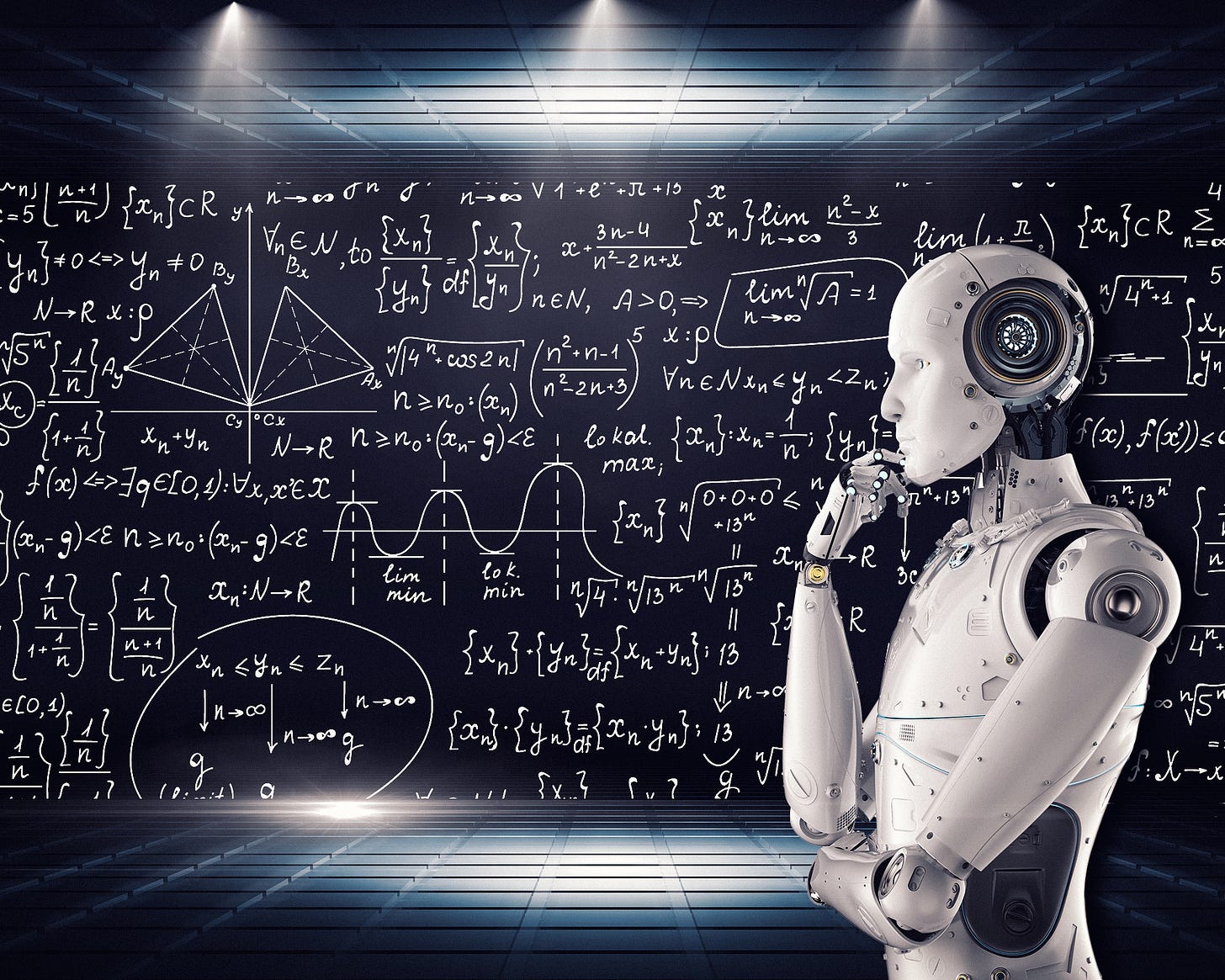
When I started writing my piece about large language models as platforms for extracting value from writing, I took it for granted that the language models themselves were doing the ‘middle layer’ of work. That is, generating outputs from inputs with nothing more (or less) than patterns extracted from training data. That training data, I knew, was scraped from publications and web sites, and represented a vast history of labour from the near and distant past. Still, representing all that text as parameters of proximity and probability, and ‘weighting’ that data towards particular outcomes, was clever stuff.
I still think there is clever stuff going on when generative architectures are built and trained. The ability to identify patterns in diagnostic images, for example, is an example of how probabilistic processing can add value alongside expert human analysis and outcomes-based research. (It may be significant that diagnosis is an inherently probabilistic activity.) But when it comes to language, I feel increasingly uncertain whether the models do the clever thing they are supposed to do, which is putting one word in front of another based on probabilistic data alone. The middle layer - between all the past text it was trained on, and user-driven production of text in the present - isn’t the model alone. It’s also an army of human beings. They are writing sample responses, reviewing outputs, assigning tags and ratings, whether this work is used to update the model or hard-coded into the interface as chat ‘rules’. This layer of labour is intrinsic to how the models generate text.
I knew that some human annotation was involved in the ‘post-training’ or ‘alignment’ phase of model development. This was a key innovation that allowed languaqe models to become usable and (supposedly) safe. But now I’ve started to follow the industry itself, rather than the user-focused hype around its products, I keep finding evidence that the human labour in the middle layer is not only extensive - more complex than labelling, iterated many times, demanding a wide range of different kinds of labour - but that it is ongoing. Developers and financiers don’t like to talk about it, but sometimes they have to, because this human labour is expensive and the costs are significant in the ‘arms race’ between the big players, and in their paths to profit.
This week, financial analysts Bloomberg and Fortune carried reports that:
contractors [from companies such as Appen Ltd and Accenture plc] are the invisible backend of the generative AI boom that’s hyped to change everything. Chatbots like Bard use computer intelligence to respond almost instantly to a range of queries spanning all of human knowledge and creativity. But to improve those responses so they can be reliably delivered again and again, tech companies rely on actual people who review the answers, provide feedback on mistakes and weed out any inklings of bias.
The focus of the article, rightly, is the conditions of these contract workers. Their pay depends on how many responses or assessments they can file every hour, as well as the ‘complexity’ rating of the work, on a scale that workers say is obscure and may be determined by an algorithm. A meta-analysis in 2022 found that crowd workers globally earned less than $6 an hour. While there are regional variations, rates of pay remain low overall because task workers can always be recruited from lower-waged economies. The work is precarious and without any benefits such as holidays, healthcare or career development. It’s also extremely stressful. Apart from the demand for speed and accuracy, it may mean assessing ‘bestiality, war footage, child pornography and hate speech’ and reviewing ‘obscene, graphic and offensive prompts’. A group of Kenyan content moderators have asked their country’s lawmakers to investigate the psychological harm they suffered in working on the development of ChatGPT.
The Bloomsberg/Fortune report also considers how these working conditions affect the ‘quality’ of the end product - that is, the outputs of the language model Bard. It notes that the workers:
say they are assessing high-stakes topics for Google’s AI products. One of the examples in the instructions, for instance, talks about evidence that a rater could use to determine the right dosages for a medication to treat high blood pressure, called Lisinopril.
Feedback loops and meaning making
That is not the kind of task you would want a crowd worker to crack out on a late night caffeine high. The prospect is marginally less frightening, though, than having a probabilistic word-picker do the same task. But TechCrunch recently revealed that Mechanical Turk workers are outsourcing many human-in-the-loop tasks to ChatGPT to boost their productivity and pay rate. With Bard now integrated into Google, a more conscientious crowd worker searching for ‘evidence’ about ‘Lisinopril dosage’ might inadvertently fall into the same feedback loop. I’m sure I don’t need to spell out the risks of using AI to check and improve AI outputs… no, I really don’t need to, because research is mounting that it rapidly leads to model collapse.
No wonder AI corporations are secretive, not just about the extent of this human labour, but about how weird, random and messy (how human) it all is. Josh Dzeiza’s brilliant investigation into the human middle layer (required reading for anyone using these technologies) found a tangle of subsidiary companies and outsourcing designed to obscure who was contracting what from whom:
Annotators are warned repeatedly not to tell anyone about their jobs, not even their friends and co-workers, but corporate aliases, project code names, and, crucially, the extreme division of labor ensure they don’t have enough information about them to talk even if they wanted to.
I think this division of labour is more than an exercise in de-skilling, de-unionising and underpaying text workers. Yes, that too, like taylorism in the factory system. But I think it is critical to the whole intellectual project of generative AI that workers in the middle layer don’t make too much sense of what they are asked to do. The doctrine that large language models have some ‘emergent’ properties that are akin to ‘intelligence’ or ‘meaning-making’ depends on their patterns and connections being purely statistical ones. For this to be true, the work of adding tags, assigning values, writing examples and rating outcomes must be treated as just another layer of statistical input. If this work is meaningful - if the data workers try to connect their piece to (what they understand to be) the whole project - if the ‘objectivity’ of their input is compromised by what they know or guess of its meaning - then the magic starts to evaporate.
Complex but meaningless task rules are not good for data workers’ mental health. With no big picture of the projects they are working on, and no formal contact with other workers as colleagues, job satisfaction is often low. Data workers’ unions are beginning to organise against the most exploitative practices, however, and projects such as Rest of World report regularly on data workers’ campaigns. Even without such conscious acts of solidarity, data workers often use their knowledge of AI systems to guess what tasks will pay highly and, according to discussion forums, it is common for them to share this knowledge. Some even share scripts that can query platform algorithms for the best tasks or task outcomes. One worker on HackerNews describes how:
‘Amazon put up a bunch of very lucrative test jobs, but you only got paid if your answer matched the majority. The majority were automating, so unless you used the sometimes incorrect answers [from the automated system], you got nothing.’
The fact that the middle layer is made up of thinking, meaning-making, motivated human beings makes it unreliable as a probabilistic function. Bad feedback, strange loops, biases and wrong information are just some of the effects that can propagate here. There is even evidence emerging (though it is early days) that GPT-4 may be getting less accurate, perhaps due to the extent of ‘post-training’ on human input. But we can’t be sure, as none of this is openly discussed.
Students and graduates in the middle layer
I previously recommended Mary L Gray and Siddharth Suri’s book Ghost Work as a primer on labour in the middle layer (though it was published before the current GenAI surge). I’ve now found a summary of key points from the book on the OECD web site. Something the authors don’t explore in depth is the age profile of crowd workers, so for this I turned to the International Labour Organisation (ILO). Their 2022 report ‘Crowdwork for Young People’ highlights that people under 30 are far more likely to be employed in platform data work. I can’t find any current evidence about students (links welcome), but a 2016 survey of platform workers (UK and Europe) found that about 10% of them were students in higher education. This was seven years ago. Considering the steep rise in the numbers employed this way, as well as the increasing numbers of students taking paid work around their studies, it seems reasonable to think that many will have experienced this kind of work before they graduate.
And after that? The EU estimates as many as 50% of workers will earn at least some of their income through platform work by 2030. The ILO study found that crowd workers were likely to be graduates, and about a quarter had masters degrees. In the same study, level of education was ‘a negligible factor in determining crowdworkers’ earnings’ but did relate negatively to job satisfaction. In other words, a lot of graduates are likely to end up working in the platform economy, many on AI related projects, and feeling less than fulfilled. A 2022 study of annotators in India, where many platforms are located (for access to English speaking graduates with lower wage expectations than in Europe or North America) concluded that ‘data annotation is a systematic exercise of power through organisational structure’. The need for ‘high quality data at low cost’ conflicted with ‘the annotators’ aspiration for well-being, career perspective, and active participation in building the AI dream’
When educators are told that we need to get across GenAI in order to keep up with our students, this almost always means ‘students as users’. But what should we say to students who are already caught up in the GenAI machine as producers, or who anticipate this future for themselves? More important, perhaps, what can we learn from them about their experiences, hopes and fears around data work? Do we accept, on behalf of students, the extreme division of intellectual labour demanded by the AI corporations, with just a few ‘original’ content producers and system designers at the top, and a multitude of text maintenance workers servicing the system underneath them? Or do we help students to investigate and understand this new factory system? Can alternative arrangements of labour be hoped for? Is it possible to dream beyond or outside of or against the ‘AI dream’?
Automation is not an inevitable outcome. It is a way of restructuring and disciplining future labour. We should all take comfort from the fact that the prophets of world automation can’t even automate their own product.
Insightful piece, thank you.
Thanks, Helen. I'll steel myself to re-immerse in the ed tech world!