I was talking to a builder friend about robot plasterers, something he’s thought about quite a lot. As you can see from the video, robot plasterers don’t exactly replace builders, but they can turn one the most skilled trades in the business into shovel-work. For some years now, they’ve been doing that in predictable settings like industrial-scale building sites with everything brand new. Gradually they are getting better at dealing with uneven walls and unpredictable settings too (that’s the ‘AI’). But there are no breakthroughs. Robot plasterers are just getting incrementally better or, depending on how you see it, the work is getting incrementally worse. The magic of AI is not making workers disappear but turning them into shovellers.
My friend knows a bit about building, and the non-magical advance of robot plastering. But when it comes to scientific research, he is convinced that AI is making everything ‘exponentially’ better. He is a well-read and thoughtful person, so when he says something like this I reflect just how much power ‘AI’ gains from crossing boundaries. In fact, this may be its one truly magical power. In our own area of know-how we see the limitations, we worry about the implications, we tune in to experts making cautious and well-evidenced remarks. But when we listen in to what’s happening nextdoor, we hear the magic show in full exponential swing.
The question of whether AI models are indeed making science ‘exponentially’ better is one I tried to address in an earlier post Never mind the quality, feel the speed. I’m not a natural scientist, but after speaking to some, and reading as much as I could, I concluded that AI - or more specifically machine learning - was not really doing science more, or better, so much as it was structuring scientific work differently:
‘Scientific models are always embedded into complex workflows, involving a range of methods, instruments, and expertise. And the outcomes are constantly checked against (real world) experimental results.’
Of course machine learning produces new results. And some of those results are important. As statisticians like to say, ‘all models are wrong, but some are useful’. But it is (surely) the task of science, and the governance of science, to determine which are useful, for whom and why, taking into account wider systems of scientific research and wider discussions about value. It is not the task of the corporations that have the most to gain from building and owning models.
Advancing humanity by 800 years
I was provoked to return to this topic by one of the key takeaway messages from the (2024) Stanford AI Index report, a project I have relied on in the past for reasonably sober analysis. Two projects were cited as standout cases for the claim that science keeps getting better.
AlphaDev’s contribution - a single step reduction in a particular sorting algorithm - is fairly niche. I followed some hacker threads about it here here and here, and the main takeaway seemed to be ‘much fuss about nothing as regards the improvement of sorting algorithms’, but some advances in AI optimisation of code. It’s more computer science than science. But GNoME (Graph Networks for Materials Exploration) does seem like the real ‘exponential’ deal.
Back in November 2023, a group of scientists published a paper in Nature that described using DeepMind’s specialist GNoME data model to find new crystalline structures from existing crystal data. They claimed to have identified 2.2 million new structures that were relatively stable, and so in theory might be candidates for new materials with real-world uses. Shortly after, a second group of material scientists claimed to have synthesised 43 ‘novel compounds’ from the GNoME candidates using a laboratory staffed by AI and robots. DeepMind and its press pack did not hold back on the excitement:
Then in January a third group of material scientists wrote a paper that challenged the findings of the second paper, and disputed many of the claims of the first.
We discuss all 43 synthetic products and point out four common shortfalls in the analysis. These errors unfortunately lead to the conclusion that no new materials have been discovered in that work.
Shortly after, a careful examination of the original claims by two members of the American Chemical Society reported:
scant evidence for compounds that fulfill the trifecta of novelty, credibility, and utility. While the methods adopted in this work appear to hold promise, there is clearly a great need to incorporate domain expertise in materials synthesis and crystallography.
Domain expertise. Ouch.
The ‘debunking’ scientists are not claiming that GNoME does nothing new. They agree that GNoME’s statistical model of ‘stability’ could be used to narrow down the number of candidate molecules to be tested in the laboratory. They emphasise that the science of making and discovering new materials is ‘tedious’ and that computation should be used to target experimental resources more effectively. But the statistical model in question made assumptions that proved unreliable for predicting stable compounds in the real world (‘all models are wrong…’).
And even with better underlying assumptions, the model produces a paradox. The bottleneck in finding useful new materials is not in discovering theoretical ones, but in testing the long list of potential candidates that was around before any Alpha got involved. GNoME has now massively extended that list, then reduced it slightly (using stability calculations), but it is still an awfully long one. And nothing on the list of theoretical molecules can find a use without an understanding of what ‘use’ means in existing contexts of human activity, without an appreciation of ‘value’ as how science gets embedded into those activities, and without hours of laboratory making and testing in what is, after all, the resistantly material world. In other words, without science.
Data (instead of) science?
Whatever the rights, wrongs and usefulness of a particular model of ‘stability’, the materials scientists who doubt the ‘exponential discovery’ claims of GNoME are not against building and training models, but in favour of a larger vision of science as ‘the making and testing of hypotheses’. Science here is a relationship of thinking people to the material world. Aspects of that relationship might be mediated via data and data models, but other aspects are mediated by theory, experimental practice and experience, visual representations that give an aesthetic grasp of material structures, and even what these authors were so bold as to call ‘intuition’.
One of the founders of artificial intelligence, and an early experimenter in facial recognition , Woody Bledsoe, put the case for AI bluntly: ‘in the long run, AI is the only science’ (cited in Machines Who Think). Bledsoe’s hubris anticipates by some 40 years Chris Anderson’s famous claim that, thanks to data-based AI, ‘science can advance even without coherent models, unified theories, or really any … explanation at all’. Science is data. Or, more accurately, where science was, there data shall be.
But science is not only method, let alone this one method of finding patterns in pre-existing data. It is a complex set of social arrangements for sharing knowledge and resources. Current ways of ‘doing science’ are far from ideal, as my friends in Science and Technology Studies would want me to point out, but since we can agree that science does matter to our social arrangements, public trust or distrust in science does make a difference to public health and other outcomes, so changes in how science is carried out have implications for us all. And if we were looking to make science more publicly accountable and trustworthy, would Google DeepMind be the first organisation that sprang to mind?
Let’s consider another celebrated DeepMind project, AlphaFold. This model has been predicting the structures of protein molecules since 2018, a capability described as ‘accelerating research in nearly every field of biology’. The (theoretical) structures produced by AF - 200 million of them so far - are essentially spatial graphs, the kind of representations you’d expect statistical models to be good at producing. But is this biology? Launching Isomorphic Labs as the commercial arm of AlphaFold in 2021, CEO Demis Hassabis suggested that yes, it is, if biology is understood as a branch of information science:
So far, no ‘isomorphic mapping’ has been found between the 2 million theoretical molecules identified by AlphaFold and real-world molecules with significant biological or pharmacological properties. Analysis of the AlphaFold claims in MIT, Nature and Chemistry World have been cautious. The MIT study found that AlphaFold’s ‘predictions performed little better than chance’, while Chemistry World maintained that:
It is very, very rare for knowledge of a protein’s structure to be any sort of rate-limiting step in a drug discovery project! … The protein’s structure might help generate ideas about what compounds to make next, but then again, it might not. In the end the real numbers from the real biological system are what matter.
Looking for some real numbers, perhaps, Isomorphic has just signed a deal with pharma companies Lily and Novartis to develop a few candidate molecules as potential treatments for unspecified conditions. It’s not exactly a ringing endorsement. Big pharma is offering big tech relatively little upfront, with the rest of the cash being linked to ‘future milestones’ and the potential to ‘exploit royalties’. So far, AFs predictions have proved at best equally good as the findings from experimentation, all of which can all be accessed from the 50-year-old, free, open and publicly available WorldWide Protein DataBank.
GNoME and AlphFold have made breakthroughs in modelling the theoretical structures of complex molecules, and this is not a trivial achievement. Natural science carries on with the work required to find out whether these theoretical models produce useful predictions and useful solutions (and working out when the models get it wrong). But the press releases and the public-facing DeepMind ‘research’ pages tell a different story. A story of these scientific steps magically completed: malaria cured, bees saved, arthritis averted, plastic waste digested.
One link from the ‘research’ pages of AF, for example, is to the excellent Drugs for Neglected Diseases Initiative. DNDI mentions that its team were invited to ‘trial’ the AF database back in 2021 and that this ‘could help to speed up development of a promising treatment’ for Leishmaniasis. However, the new molecule that DNDI is currently trialling for this disease was actually discovered much earlier. Under an invitation to ‘meet the millions of researchers using Alphafold’ we read at least several ‘AlphaFold stories’ showing AF data used in conjunction with experimental data to produce promising future applications. Some, not so promising. A paper cited in support of AF modelling of enzymes, for example, actually reports the use of experimental techniques: AF does not appear in it anywhere.
The future and conditional tenses get a thorough workout in these promotional pieces. Might, could, would, if, when. The same tenses are often well used in the introduction and discussion sections of peer-reviewed scientific papers - the parts where authors are invited to speculate beyond the limits of their own evidence. Potentially. Theoretically. Further research could clarify. In 2021, when AF first unveiled its 2-million-plus gift to science, material benefits could reasonably be projected into the future. But it’s 2024 now, and the ‘exponential’ possibilities should be starting to lift off. Where are the significant advances in science, rather than in modelling methods? When are science writers and journalists going to start doing some work on these claims in the more exacting environment of the present tense?
And what resistance is being offered to the displacement of science by data, the colonisation of science journals by papers on machine learning? Just about every serious study of the topic finds technical limitations. One is data leakage, which has been found in nearly 700 machine learning papers across 30 fields of natural science (and counting), in every case leading to overestimation of the model’s accuracy (‘once corrected for data leakage they did not perform any better than older methods’). Another is the problem of using ‘blackbox’ models as keystones of scientific method, meaning that reproducibility and accountability are lost. Scientific discovery in the age of artificial intelligence, a grandstanding prospectus published in Nature last year, with researchers from DeepMind and MicroSoft among its authors, conceded that:
‘Minor variations in implementation can lead to considerable changes in performance… AI approaches can suffer from reproducibility due to the stochastic nature of model training, varying model parameters and evolving training datasets’
What solutions do these authors suggest? Better models: ‘standardized’ methods, official benchmarks, more data, more AI experts on the team. Solutions that just happen to favour the largest models and the organisations that own them. Even the epistemological problems - the same paper notes that AI models lack causality, generalisability and ‘theoretical guidance’ - are deemed to have technical solutions that have just not been worked out yet.
But epistemological problems are not methodological details. They represent a significant break with established scientific thought and values. Unexplained, proprietary models used in methods mean that potentially important work is no longer transparent, open, reproducible or fully peer reviewed. These are not fashionable, woke refinements to the practice of science; they are literally its foundation. Safety and ethical concerns about AI - its biases, inaccuracies, environmental and labour costs, the delusions it produces - these all demand a debate about what science owes to society. Computational fixes do not provide the answer.
In fact, a recent perspective piece in Nature suggested that scientists may be particularly susceptible to certain AI illusions:
scientists who trust AI tools to overcome their own cognitive limitations become susceptible to illusions of understanding… The proliferation of AI tools in science risks introducing a phase of scientific enquiry in which we produce more but understand less.
You would think this delusion/proliferation is a new problem. But it has beset science from the start. In mid-eighteenth-century Europe, the sheer productivity of the new science was felt to be running ahead of any capacity to reason about and benefit from its discoveries:
‘The desire to know is often sterile because of an excess of activity… silence on those who only swell the volume of science without increasing its treasure … We would then free so much space in our libraries!’ Entry on ‘Criticism’ in the Encyclopédie, cited by Marina Garces in New Radical Enlightenment
The challenge that data-as-science presents to science-as-understanding is not new, but it is newly powerful. If biology is information processing, as Dennis Hassabis has argued, and if computer models do information processing best, is there any need for ‘understanding’? Is there anything, really, that biological science and biological scientists can bring to the party?
Follow the money
Models can be wrong/useful in different ways, but data is always valuable to somebody. And I think the glamour of ‘AI’ and ‘machine learning’ are being used to distract from the fact that this is still, essentially, about extracting value from data. In fact, I would argue that modelling shifts the balance of power even further towards the players with the most data.
When the AI surge began to take off in 2022, critical data science had made huge inroads into academic thinking and the public imagination. (Rather than trying to summarise the work of many brilliant scholars, I’ve included a reading list below.) The harms and injustices of big data was so widely understood that by the early 2020s there were not only dozens of academic courses on data justice issues, but many books for the general reader too. Highly publicised abuses such as the Snowden revelations of mass surveillance and the Facebook-Cambridge Analytica scandal added to the sense of public dismay. The entry on Science and Big Data in the resolutely mainstream Stanford Encyclopaedia was amended to include a lengthy discussion of big data (in)justice, and how data at scale tends to give undue influence to a few big, data-intensive projects and institutions.
In a direct rebuttal of Chris Anderson’s ‘end of theory’ post, philosopher of science Sabina Leonelli wrote that big data in biology, far from providing a neutral space for disinterested exploration:
turns out to represent highly selected phenomena, materials and contributions, to the exclusion of the majority of biological work. What is worse, this selection is not the result of scientific choices, which can therefore be taken into account when analysing the data. Rather, it is the serendipitous result of social, political, economic and technical factors, which determines which data get to travel in ways that are non‐transparent and hard to reconstruct by biologists at the receiving end.
Cue the arrival of ‘AI’, which is very quiet indeed on the subject of data and its ‘travels’, but loud on the magic of ‘finding patterns’ and ‘producing new discoveries’ from data. If data is mentioned at all, it is as a kind of raw material for ‘intelligence’ to consume. Never mind the data, feel the algorithms. But AI models are still big data: in fact, they are the biggest data. Data that has been acquired, amassed and tokenised at a previously unthinkable scale. Data that has had advanced machine learning techniques applied, across many computational layers, to produce something really bigly big. (A recent report on the advances in AI since 2012 concludes that scaling up computing power and data processing capacity has been significantly more important than the advances in computational method.)
Big data, as noted, has many flaws, but in its untrained state it at least allows for users with the requisite know-how to apply different interpretations and analytical methods. Experts might still reconstruct where the data has come from and what its qualities are. The AI model, on the other hand, is singular, proprietary and closed. It fixes the relationships among data terms into a complex but unyielding form: the form that produces the ‘best’ outcomes for a particular kind of analysis, as determined by the model’s developers. This form resists explanation, not only of the relationships between data terms, but of the model’s own complex construction. When large language models replace search results with a single summary, this is the bargain they are offering: one unquestioned result over many interpretations. This is the bargain on offer from machine learning models in science too: instant correlations over the tricky process of thinking, questioning, arguing, and counter-arguing.
In the ‘material world’ that Madonna sang about, the world ruled by money, statistical modelling concentrates economic power as well as data. Data for GNoME was drawn from the Materials Project, an essential resource for research of both experimental and computational kinds.
Findings are more valuable to science when they are shared. But the more value is concentrated in the data, the more power it has to shape research, research careers and research funding. And when new commercial players such as Google DeepMind come on the scene, this fragile sharing ecology is disrupted.
Scientists who had contributed to the Materials Project were not all happy (as reported in Wired) that the GNoME model had ingested their data into a proprietary model, with its potential for commercial use.
(Pushmeet) Kohli is Vice President of Google DeepMind, a shotgun marriage between DeepMind and Google’s Brain division, explicitly to put Alphabet/Google back on terms with MicroSoft/OpenAI in the AI race. So Kohli has a lot of investments riding on this project. Notice that what ought to be a deal-breaker for science - the lack of a clear, explainable or reproducible method - is justified by commercial interest. Notice that although DeepMind regularly contributes to scientific papers on modelling, there are ‘no plans to release the model’ for other computer scientists to evaluate.
As the deal between Isomorphic and Novartis shows, somewhere down the line these models are expected to generate commercial value. Which in frank terms means that Google/Alphabet hope to get paid every time someone uses a product or innovation that may have had its development accelerated by access to an Alpha model. Elsewhere in the Alpha stable, every possible single base mutation in the human genome has been modelled, a development that has had little impact on genomic science as yet: (‘the improvement over other algorithms is modest’) but great potential to capitalise on any single base mutation that may in future be found significant to human health. The AlphaMissense project claims to have partnered with Genomics England to test its predictions. Genomics England, repository of the genomic data of more than 100,000 NHS patients, records no such partnership on its web site. However, Genomics England is included in Palantir’s proposed ‘National Technical Framework for Life Sciences’, through which the company offers to ‘differentiate the UK in the global healthcare and life sciences market’ by selling access to the uniquely valuable data from UK healthcare records. Surely only the hardest of cynics is worried by these developments.
As I argued at the start, AI restructures the work of science. The Nature paper on the coming age of machine science outlines how ‘research teams will change in composition to include AI specialists, software and hardware engineers’. The same article notes that ‘the computational and data requirements… are colossal… As a result, big tech companies have heavily invested in computational infrastructure and cloud services… [There are] new modes of industry–academia partnerships, which can impact the selection of research questions pursued.’ A recent review of the relationships among machine learning, data and research concluded more simply: ‘academia needs to prioritise feeding the machines’.
Just as the purveyors of large language models have been wooing the publishers of valuable content into partnerships, so Google is wooing research centres that own valuable scientific data, whether this is plant DNA, human healthcare records or robotics. I have no doubt that some scientists are finding good uses for the Alpha models, and that smaller, more specialist models are speeding up parts of the science workflow. But I think the claim that AI is accelerating science deserves far more critical examination, and the potential for the work of science to be disrupted and redirected by new consolidations of data and capital should be part of that conversation.
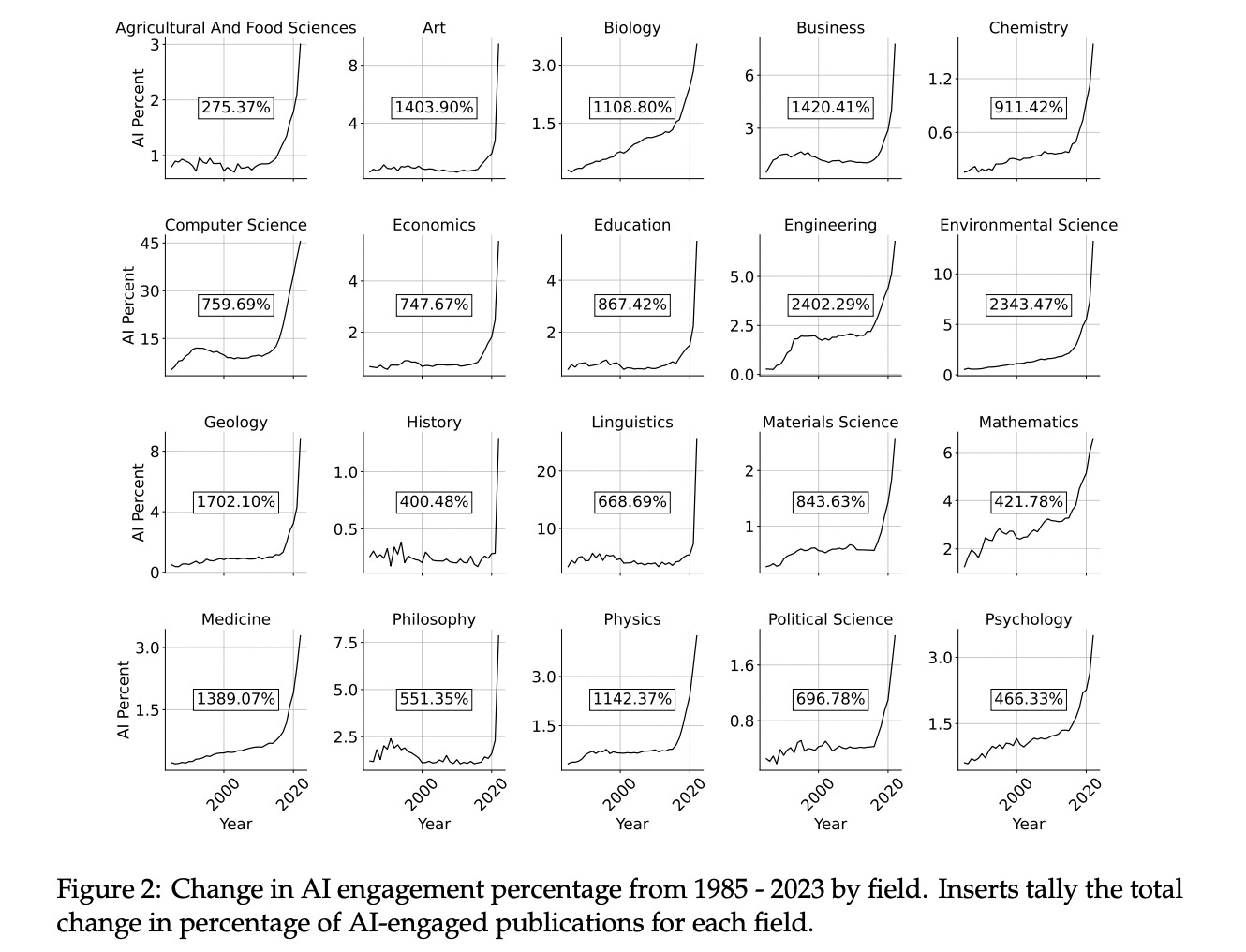
Science may not be expanding exponentially, but science’s love affair with AI is lifting off at a magnificent angle, as shown in these graphs from Duede et al (2024). Science is becoming more data-driven, more capital intensive, more oriented towards research questions that can be answered using machine learning methods (more correlation, less explanation), and more cosily in bed with big tech. And there, in bed with its Alpha, we must close the door on science and tiptoe away. Perhaps bigger really is better. Or perhaps, as Madonna once sang, the boy with the cold hard cash is always Mr Right.
Critical data studies
A reading list in date order, open access wherever possible. For an up to date list of critical data/tech initiatives, start with Ruha Benjamin’s resources page.
Critical questions for big data: Provocations for a cultural, technological, and scholarly phenomenon, Boyd and Crawford, 2012
Towards critical data studies: Charting and unpacking data assemblages and their work, Kitchin and Lauriault, 2014
Data colonialism through accumulation by dispossession, Thatcher, O’Sullivan and Mahmoudi, 2016
Invisible Women: Perez, 2019
Good Data, Daly, Devitt and Mann, 2019
Digital dead end: Fighting for social justice in the information age, Eubanks 2011
Data Feminism, D'Ignazio and Klein, 2020
Data Justice (link is to book contents only), Dencik, Hintz, Redden and Treré, 2022
Epistemic Injustice and Data Science Technologies, Symons and Alvarado, 2022
Data ableism, Charitsis and Lehtiniemi, 2023