It’s a week since the new Labour Government was elected in the UK, and I don’t have any inside track on their ‘artificial intelligence’ policy, but policy there will be, and a whole new Minister for AI to see to it. Matt Rodda has so far said little in his new role but is on the record at the last Labour Party Conference stating that ‘AI will be a strategic priority’.
In the short space before we learn what that strategy priority will look like, I am taking my soundings from two main sources: Tony Blair’s piece in The Times on Saturday, much of it reiterated in his speech to the Future of Britain conference, and Peter Kyle’s visit to Silicon Valley in February of this year. There are other influences on the Starmer government, such as the more measured approach of Labour Together, but these two seem most likely to set the tone on technology policy.
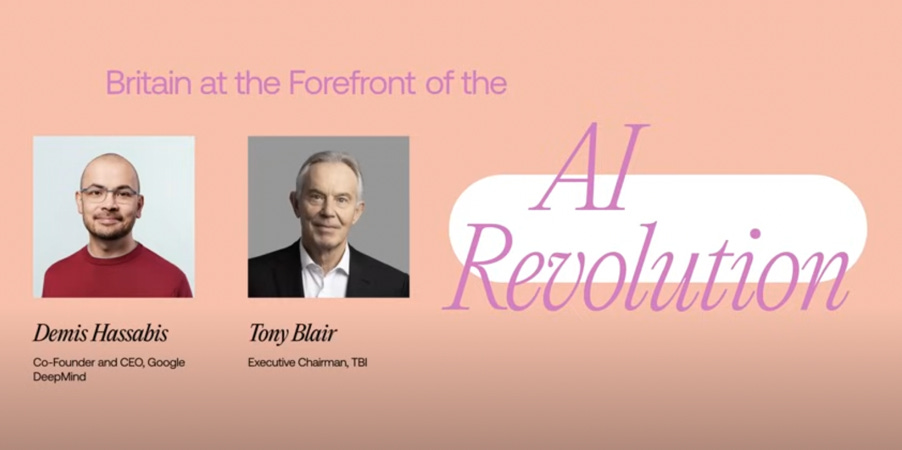
It’s an open secret that the Tony Blair Institute for Global Governance (TBI) has been advising Starmer for years, and its 1000-odd staff will be filling key advisory roles in his government. TBI’s relentlessly millennial vibe is technology on drum and privatisation on bass, with backing vocals from a band of states with dodgy human rights records, and Larry Ellison (tech billionaire) on the decks. So it’s no surprise to hear Blair crooning for Starmer to feel ‘the full embrace of the potential of technology’. AI is ‘the only game changer’ that will ‘turbo charge growth’ and ‘save the government tens of billions of pounds’. That’s a bold prediction when there is growing scepticism from investors and push-back from almost every commercial sector against the hype around AI productivity. But the public sector has always been a place for big tech to find acceptance when the commercial world turns harsh and judgy.
Specifically, Blair proposes to use AI to ‘cut workforce time’ by 20% across the board, amounting to around 1.15 million public sector jobs by the end of the next parliament. Expected redundancy payments of £24billion will be offset by an AI productivity bonanza, and if trade unions are pushing back against these proposals, it is surely a sign that they must be on the right track. Working with Faculty AI (spoiler alert - Faculty will appear more than once in this piece), TBI analysed roles in the Department for Work and Pensions and estimated that over 40% of tasks here could be automated. Ironically or not, ChatGPT was used in the task analysis. DWP is twice as nice for AI, it seems, because so much of its work is ‘citizen-facing interactions’, and because pensioners, people with disabilities, and people in situations of desperation and poverty are especially keen to talk with chatbots about their circumstances. (TBI/Faculty admit that the study was theoretical and did not actually interface with any public servants or service users).
I found most of the TBI/Faculty report difficult to follow, repetitive and badly referenced. Perhaps ChatGP was let loose on more than just the task analysis. In one of its more lucid suggestions, AI was:
analysing streams of real-time data to produce actionable insights at an otherwise impossible pace and scale: for example, identifying fraudulent or erroneous claims in progress and nudging citizens away from proceeding with these claims.
If only this gripping scene had not been cut from Minority Report.
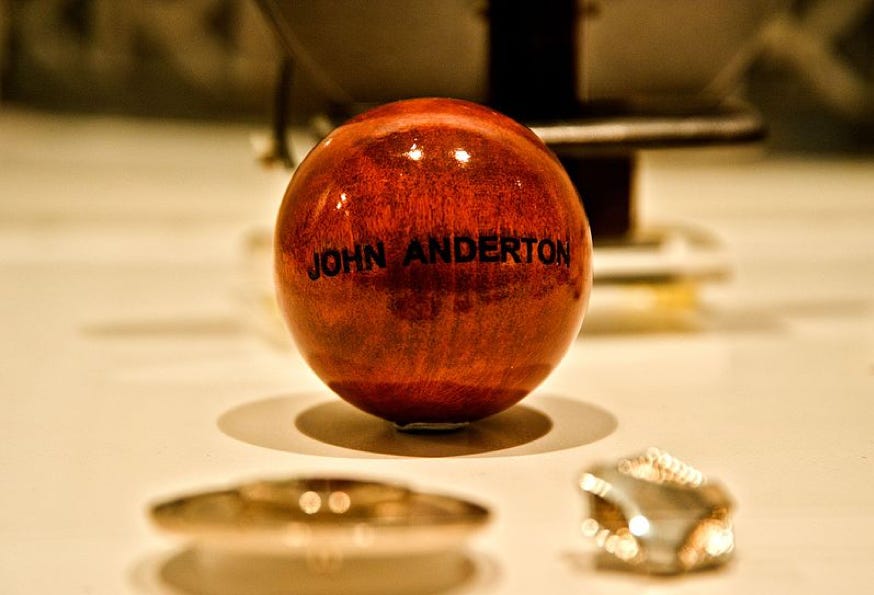
TBI’s proposals for AI are essentially the same as its proposals for public service reform (and at this point you might want to refer back to a piece I wrote last year: AI and the privatisation of everything). For every problem of infrastructure the answer is digital infrastructure, and the shibboleth for digital infrastructure is currently ‘AI’. ‘Almost everywhere, AI can help us reimagine the state’. Public transport? ’Mobility as a service’ ‘ (‘combining data and ticketing’). Unemployment, or under-employment? ‘A digital employment assistant for every claimant’. Crumbling schools? ‘A secure, functional and interoperable digital learner-ID system’. People dying on waiting lists and hospital trolleys? You’ve guessed it: ‘personal health accounts’. And most of this infrastructure will naturally be based in California, even if the people whose jobs it displaces and whose data it aggregates are living in the UK.
Let’s turn now to Peter Kyle, the new SoS for Science Innovation and Technology, and Matt Rodda’s boss. He has shown an interest in digital inclusion and promises more government support for digital skills. If that is channelled through organisations like the Good Things Foundation with its local hubs and public sector ethos, and if it means lifelong capabilities rather whatever tasks mTurk is assigning this week, that will indeed be a Good Thing. Kyle also promises a longer term approach to R&D funding, so there is a chance to build national capacity in relation to the challenges and opportunities of AI: research labs, models, development tools and data sets, and regulatory initiatives to meet the particular needs of the UK (ideally open and publicly owned).
But there are reasons to think that building these public capacities may not be Kyle’s priority, and to understand that we need to look at his relationship with Silicon Valley. After a think-tank-funded visit in February with MS, Amazon, Meta, Apple, OpenAI and Anthropic, Kyle seems to have been given a free go on the Tony Blair fantasy calculator (the one that works in denominations of ten billion), declaring that: ‘The growth opportunity from AI [ ] could see the exchequer receive £60 billion more in revenues’. On his return to the UK, he lost no time reassuring big tech that a Labour government would make few new regulatory demands: a requirement for ‘frontier labs to release their safety data’ still sounds like marking their own homework. Instead, a Labour government would ‘unblock the tech barriers’, ‘stepping out of the way to allow large technology companies to build critical infrastructure like data centres in the UK’.
How will it serve the UK economy to become a lorry park for big tech’s data juggernaut? Kyle does not address this question. Instead, he picks out two examples of AI from which he extrapolates many opportunities for the public sector: ‘faster cancer scans’ and ‘personalised lesson plans for children’. The promise of faster cancer scans also comes with a personal story that makes it very hard to ask more probing questions. Kyle tells us:
‘I have seen AI tools which I believe would have caught my mum’s cancer earlier. It is personal for me to get this technology used in a way which keeps families together for longer.’
Cancer scans and lesson plans
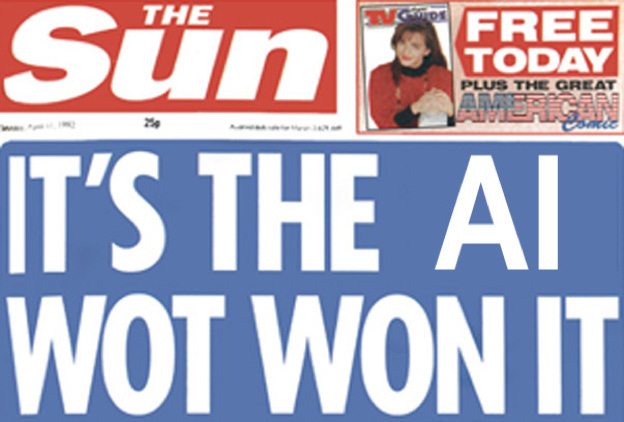
Cancer scans and lesson plans are recurring motifs in the TBI reports as well. So what do these two applications of AI have in common? Regular readers may recall my deep dive into the claims of machine learning models to be revolutionising certain fields of science. I think the claims of AI to be revolutionising cancer diagnosis and lesson planning are rather similar. You could almost call it a playbook.
In the case of cancer scans, it was 2016 when ‘godfather of AI’ Geoffrey Hinton demanded that the world should stop training radiologists because AI was making them redundant. Thankfully, the world did not listen. In fact the NHS is experiencing its worst ever shortage of radiologists right now, as training fails to keep pace with the demand for skilled diagnosticians. Hinton was right that radiological imaging would account for the majority of ‘AI’ applications in healthcare, since diagnosis and prediction are what machine learning is (sometimes) good for. But he was entirely wrong to think that radiology can be reduced to those aspects of the imaging-to-diagnosis workflow that machine learning (ML) can support.
ML systems are specialised to certain kinds of image, and sometimes even to specific imaging equipment, and even these highly specialised systems lose efficiency when exposed to real world data. So there is no generic ‘AI radiologist’ that can detect cancerous signs from a multitude of image types. Expertise is needed to interpret even algorithmically-enhanced images, and to check and sign off on any diagnosis that may be suggested. In 2021, a review of ‘AI for clinical ontology’ found that:
While there have been thousands of published studies of deep-learning algorithm performance (Kann et al., 2019), a recent systematic review found only nine prospective trials and two published randomized clinical trials of deep learning in medical imaging (Nagendran et al., 2020).
And three years further on, a major synthesis review still found that:
even though the potential of AI software to impact radiology is large, little is known about how it is changing the quality, efficiency and costs of health care…
it has been proposed by multiple parties, including the FDA [US Federal Drugs Agency], to change the way AI software is regulated from a standalone evaluation to a more systemic approach where the context of the clinical and human interaction is taken into account. This would require more real-world monitoring contributing to the available evidence on the actual clinical impact of AI on health care.
At the sharp end of health care, I was recently put on a two-week cancer pathway and waited eight weeks for surgery. I’m fine, and my care was good when I got it, but the strains on NHS resources were obvious. Most people in the UK can tell a similar story about themselves or a loved one - we all have the equivalent of Peter Kyle’s mother we’d like to be treated faster. But even if diagnosis is improved in some measure by new imaging tools, making a difference to a cancer patient’s outcomes still requires investment in oncologists, specialist nurses, lab technicians and surgeons, in operating theatres, hospital beds and therapies.
Just this week, a group of experts writing in the Lancet have begged the incoming government to stop seeing AI as a ‘magic bullet’ for cancer and invest in some of the critical infrastructure that has been so neglected. The UK is currently one of the worst of the developed nations for cancer survival rates, and unnecessary cancer deaths align closely with indicators of deprivation. But these are much bigger problems than can be dealt with by machine learning in some diagnostic workflows.
The lack of rigorous evidence for ‘AI’ in real-world cancer outcomes will remind regular readers of the lack of rigorous evidence for ‘AI in education’ that I covered in a previous post. Melissa Bond and her team concluded a meta-synthesis review in January 2024 with ‘a compelling call for enhanced rigour’. Even hotter off the press, a systematic review of the literature of ‘trustworthy and ethical AI in education’ in July found that:
‘the complete absence of pedagogical, design-based as well as empirical, evidence-based studies on experiments from practice is notable, and underlines the starting point of a scientific debate that is still lacking practical developments and implementations’.
But TBI’s Future of Learning report refuses any doubts about practical implementation. AI is set to raise national attainment and GDP by 6% ‘at the most conservative estimate’. This figure seems to have been arrived at by taking the mean average benefits reported in 14 meta studies of ‘pre-AI-era ed tech’ and multiplying by the effects on lifetime earnings found in a single study of pupils completing their GCSEs in 2001. Readers might want to check out the first few ‘references’ in this report to get a sense of the academic rigour involved. (I hope Larry Ellison thinks his funding is being well used here, though he may be distracted from considerations of learning gain by the recent collapse of his AI-for-cancer-detection start-up.)
The roadmap to achieving these incredible advantages with AI is ‘a single digital ID for every learner’, collating every source of data about their progress and achievements. Labour may have ruled out Tony’s plan for digital ID cards to manage migration, but none of the uses they have for ‘AI’ in public service is possible without data collection and integration on a national scale. In education, TBI claims that this will allow the classroom to become a ‘real-time data environment [that] would mean AI could mark a class’s work in less than a second and provide personalised feedback’.
In a familiar use of what I like to call the ‘extended conditional’ tense, the report goes on to anticipate that:
adaptive learning would become the norm in every subject, using data collated from different apps to create assignments that challenge, stimulate and engage the learner without leaving them behind. Each learner would have access to a personal learning map, with AI-supported tuition, and could revisit any content when needed’.
In Teaching Machines: the history of personalised learning Audrey Watters records the promise of individualised learning over more than a century, showing how it has often accompanied the introduction of new technologies. In this real history – the ‘extended imperfect’ if you like – educational benefits have proved elusive, inequalities have often been exacerbated, and a great deal of money has been spent. ‘Personalised learning’ almost always turns out to mean creating efficiencies in admin systems by collecting a lot of personal data, efficiencies that could be translated into benefits for learners if the savings were all invested back into teachers and learning support. But (and this may come as shock) organisations tend to have other uses for efficiency savings, and tech companies always have other uses for the data.
Still the promise refuses to die. Just last year, FacultyAI’s ‘hackathon’ for education came up with the question:
What if teachers could use GenAI to create lesson plans, homework exercises, personalised worksheets and assignments for students based on the curriculum and to support content covered in class?
‘What if?' indeed. The Oak National Academy had in fact just received several £million to develop ‘AI lesson planning’ capabilities for UK schools (nine months on, the web site shows that this is ‘coming soon’).
Data subjects and risky futures
In the use of cancer scans and personalised lesson plans to advocate for a general ‘AI revolution’ in public services, there is more going on than an ignorance of contextualised research. There is an ethos of diagnosing needs and risks instead of addressing people’s conditions of life. TBI envisages‘citizen-centric digital identity’ systems interfacing between people and services at every touch point: managing migration, policing protests, tracking qualifications and skills, monitoring healthy activities, or assessing claims for benefits. ‘Centric’ suggests empowerment, but it’s clear that the person in the middle of these converging data systems can’t be recognised as a citizen or a service user unless they submit to this systematic surveillance.
And what is government going to do with all this data? Provide more and better services? Target services differently? Collectivise the identified risks? It isn’t clear. Nor is it clear who will own, manage and enable access to citizen-centric data. Part of Blair’s thesis is to treat all data as a marketable asset. In this interview, he cites ‘bioscience and AI’ as the key drivers of UK growth and argues that the country’s greatest asset lies ‘with the NHS, because it's a single payer system, and you have got data that is of immense value to creating your bioscience industry and to letting it flourish’. So the best way for the UK to participate in the next technological revolution is to turn over its NHS and Biobank records.
The second part of Blair’s plan for data is to use it – via personalised technology – to give individual service users more ‘informed choice’. Personalised Health Accounts and genomic sequencing are classic TBI projects, as well as personalised learning accounts. They provide major new market niches and procurement opportunities for private tech, while contributing in a deeper sense to privatisation by making health or learning a matter of personal self-knowledge and self-investment. Something to be read off from expensive wristwear rather than guaranteed by a democratic state.
The subject/citizen is now a body of data, invited to know themselves as a unique bundle of desires and needs (and genetic codes), but known by the state and its corporate partners in terms of quantified risks. Whatever can be ‘personalised’ in a public service is almost by definition non-essential. So individual users are always right about what they want, but the needs people have in common can be refused any reality. Tech capital in particular has no capacity to build the foundational services people need, but can help to manage those needs, providing the interface between citizens and what remains of the common good in the form of ‘choices’, ‘customer services’, ‘personalised plans’ and ‘diagnoses’, chatbots and AI-based apps. Meanwhile the state can use all that data to provide a kind of risk management service or insurance back-stop to private capital as it moves into the public sphere. Calculating risk is exactly what deep learning is good at.
Regulatory capture
When it comes to AI, there is also the question of its own risks and how a Labour government might seek to manage them. The manifesto promised to criminalise the production of deepfake images, as the last government did. End Violence Against Women has argued that this would require thousands of individual prosecutions to achieve any deterrent effect. There is no suggestion that the tech companies supplying the means to create and spread harmful images should be subject to any legal sanctions. But ‘outlawing nudification’ is at least a statement of intent.
Beyond deepfakes, Peter Kyle has criticised the last government for being ‘too slow’ on AI regulation overall. And it’s true that Sunak was reluctant to place any constraints on the sector that he rather obviously hoped would employ him in the very near future. His Frontier AI Taskforce was so stuffed with SV insiders that a 2023 House of Lords Inquiry into Generative AI went so far as to express concern about regulatory capture.
(What is regulatory capture? A recent article in Policy and Society traces the deep influence of big tech/big AI across multiple domains of public policy, describing the biggest corporations as ‘super policy entrepreneurs’ :
semi-autonomous and semi-sovereign entities enjoying considerable global authority. Sovereign states are also beginning to treat Big Tech like sovereign actors. Governments worldwide have started assigning diplomats to work exclusively with Silicon Valley.
Silicon Valley, of course, has long been assigning its own diplomats (lobbyists) to ‘work with’ state legislators. A new report from CommonWealth identifies other mechanisms of influence: using venture capital to monopolise R&D; funding thinktanks; capturing AI talent; and influencing (keynoting, hosting, sponsoring) major conferences and policy forums.)
Surely these efforts will continue under Labour. But will the outcomes be any different? Chris Bryant, who now has responsibilities for cyber and digital, said in opposition that: ‘
A Labour Government would introduce binding regulation of the most powerful frontier AI companies, requiring them to report before they train models over a capability threshold, to conduct safety testing and evaluation and to maintain strong information security protections.
‘Binding’ sounds tougher than the laissez-faire approach of the last government. But it still asks AI companies to self-report their approaches to training and to safety. It still limits regulation to ‘the most powerful frontier AI’. ‘Frontier AI’ is a term invented by leading AI companies and defined as ‘highly capable foundation models that could possess dangerous capabilities’: both models and dangers are therefore still in the future. The ‘frontier’ companies have lobbied hard for self-regulation with some kind of government oversight, creating strong partnerships between the current biggest players and any future regulatory bodies, and making it much harder for models to legally be developed by anyone else.
For these companies, the Labour manifesto could not be more reassuring:
We don’t seek to disrupt the voluntary code, but we will certainly make sure [the standards] are maintained and that any new entrants into the market will know that there’s a legislative foundation that must be adhered to.
Labour also seems happy not to disrupt the AI Safety Institute, that advocates an extremely narrow and technical approach to safety, ignoring risks to the environment, knowledge economies and human rights. The Institute’s safety regime looks exactly like the kind of red teaming that already goes on in Silicon Valley, and Matt Davis of AI Now argues that this risks the Institute ‘essentially becoming the provider of voluntary services to large incumbent companies’. So it may be significant that while the Institute is still ‘acquiring the necessary technical expertise’ in the UK, it has already opened an office in San Francisco for ‘conducting joint evaluations of AI models’ with key players over there.
This surely is what CommonWealth meant by the capture of expertise.
Meanwhile Peter Kyle has given numerous speeches to business leaders promising to ‘cut red tape’ on AI start-ups and innovators. The ‘Regulatory Innovation Office’ that he proposes to ‘speed up’ the Government’s response to AI developments is meant to accelerate innovation, not regulation. Nor is Labour immune from more direct forms of capture. Faculty AI have donated £36k this year to ‘work on AI policy research’ in Kyle’s personal office. Yes, it’s Faculty again! Developed for their core business in defence, border control and cybersurveillance, their Frontier AI platform (nice name) has been adapted to support operational decision making in the NHS.
Faculty received £350k to consult on AI use cases in education, as previously covered here, and the company now provides safety testing services to the AI Safety Institute, since its role as data partner to the Vote Leave campaign gave it unique insights into the interface between politics, privacy, and data safety.
So, far from challenging the previous government’s trajectory, Labour seems even more keen to open up UK public sector to AI based services. People who have objected to AI spy firm Palantir running the NHS data service are chided by Labour’s new Health Secretary, Wes Streeting, as ‘the tinfoil hat brigade’, though they include health unions, patient groups, privacy campaigners and well-known tin-hatters the British Medical Association. Streeting promises to ‘fight’ such nonsense. Better use of data will be ‘equivalent to hiring thousands of new doctors’, he claims. (Readers, I checked the 2022 report he referred to, and the main takeaway is that doctors are fed up with NHS IT). In the same speech he chides patients who ‘still wait on the phone at 8 am, or even queue up in person in the cold on a frosty morning just to see a doctor’ when they could be using the NHS App. I use the NHS App myself, and I can’t help feeling that the problem is a lack of doctors at the other end of whatever tech you are using, and not a lack of tech. (Can I resist mentioning here that Google Deepmind tried and failed to build an AI-powered NHS app some years ago? No, I don’t think I can.)
It’s true that public procurement of corporate IT systems has not been an unqualified success in the UK, perhaps most spectacularly in health (oops, that was one of Blair’s). And it’s true that data systems are not at all well integrated, one consequence of splitting up what was once a national health service into competing entities, much as local authority managed schools have been split up into competing academy chains, and public providers into private water, power and rail companies. But is the solution bigger data management contracts? Is the solution ‘AI’?
What to build?
Perhaps it’s time to admit that the only thing keeping these disparate state services together are streams of data. Not a universal ethos of state care ‘from cradle to grave’, and certainly not a commitment to accountability, with representative bodies deciding how services can better meet the priorities of today and of the future. You could point instead to the plethora of regulatory bodies that have been set up in the wake of privatisation, bodies that have the tricky job of promoting public interest and safety with companies whose first statutory responsibility is to their shareholders. But they too rely on data to monitor what is decreed to be the public interest. They do not require an actual public with an actual voice.
So Labour is promising a new National Data Library, bringing together government, industry and university data sets, and using them to deliver its vision for ‘data-driven public services’. Whether this can work in the public interest depends very much on who gets to build, own and manage the magic mountain. If Kyle wants to ‘reduce red tape on AI innovation’ to ‘support the next 10 DeepMinds to start up and scale up here within the UK’, he might want to start by asking why DeepMind has been owned by Google for the past decade. Or why MicroSoft has just hoovered up InflectionAI, or why the UK’s most innovative chip maker, Arm, is now owned by SoftBank and traded on the NASDAQ. One reason the EU is so keen to regulate hard on AI is to ensure home-grown businesses can compete with the US giants, and to keep on shore some of the employment opportunities, intellectual properties and tax take. This is only possible with the kind of public investment that Labour seems to have ruled out, relying instead on deregulating in ways that incentivise the private sector.
And so we return to the one concrete promise in the manifesto, to ease planning restrictions on big data centres so that Californian tech companies can capitalise on the relatively cool, well-watered environment of the UK. Will these data centres be part of a sustainable energy transition? Will they power up UK businesses and public services? Or will they, like Amazon warehouses, benefit from low regulation and low-paid workers while taking the power, profits (and tax liabilities) back home? It’s worth noticing that the decision by ScaleAI to site its European headquarters in London has been trumpeted by both incoming and outgoing governments as a sign that the UK is an AI world leader. But ScaleAI is not another DeepMind, pushing the boundaries of research and development. It is a data services company, supplying cheap labour from India, the Philipines and other parts of the Global South to annotate training data. It was named by the Oxford Internet Institute in a study into poor labour practices. The new government will quickly have to decide whether it is an asset or a liability that the UK is at time of writing the least regulated nation in Europe for labour rights and AI safety.
If it is time to build, who is building, and how does it benefit workers and citizens? As Matt Davis argues later in his excellent article for AI Now:
Instead of assuming that any and all types of AI will produce economic growth and societal surplus with minimal state intervention, government needs to develop a clear articulation of what “public benefit” looks like in the context of AI and what sort of AI sector will deliver it. It also needs to understand how AI… impinges on other long-term priorities such as environmental obligations and the concentration of power in the digital economy.
Only governments can do this. But if governments are captured by big AI, they are less likely to take action on risks such as environmental degredation, the concentration of power, or labour rights. And regulatory capture provides a different lens through which to view Kyle’s visit to Silicon Valley in February, a visit funded, hosted and largely scripted by big tech. We should perhaps stop asking what policies the Labour government has in mind for AI, and ask more pertinently: what policies does AI have in mind for the Labour Government?
Referred here by John Naughton and got a compelling set of insights as the reward
As ever Helen a very thought provoking post. Thank you for writing such an in depth reflection.